Enterprises need access to data-driven insights faster than ever before. Analytics use cases have evolved from traditional, precanned reports to self-service and guided analytics. Enterprises exploring solutions to these challenges are steadily embracing the cloud data warehouse. These platforms provide agility, scalability, and simplicity that enable the enterprise to focus on data solutions rather than spending valuable effort on peripheral overheads.
Advisor
Cloud Data Warehouses: A Paradigm Shift in Data Platforms
Posted August 26, 2020 | Technology |
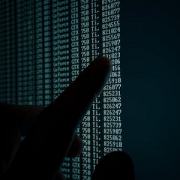
Don’t have a login?
Make one! It’s free and gives you access to all Cutter research.