The role of data architects is sometimes vaguely defined and tends to fall on the shoulders of senior business analysts, data scientists, or database and ETL specialists. As with any kind of architecture, designing for uncertainty is a key requirement with data architecture.
Advisor
A General Recipe for Creating Data Architectures
Posted February 24, 2021 | Technology |
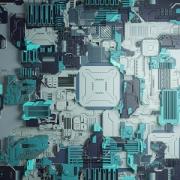
Don’t have a login?
Make one! It’s free and gives you access to all Cutter research.