In a world increasingly dominated by artificial intelligence (AI) in its various guises, the danger of good data going bad — what we might call “data rot” — is becoming pervasive and dangerous. In this fourth Advisor of my series, I consider a couple of real — and possibly unexpected — ways the rot can set in.
Advisor
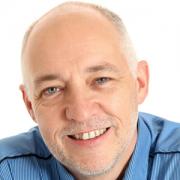
Don’t have a login?
Make one! It’s free and gives you access to all Cutter research.