There are particular business behaviors that actively poison data or degrade data quality. Although not their intention, it’s the direct consequence of how these activities are performed. This Advisor takes a close look at the concept of self-service and how it contributes to the problem of data poisoning.
Advisor
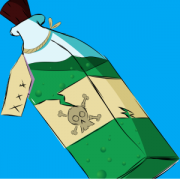
Don’t have a login?
Make one! It’s free and gives you access to all Cutter research.