Cutter Consortium Senior Consultant Curt Hall presents intriguing examples of how corporations and governments use AI and compatible technologies to move us toward a more sustainable world. He explains how many companies are using AI to reduce the energy consumption of … AI(!) and other digital technologies. He illustrates innovative technology-based solutions being developed for tracking carbon emissions and presents a selection of companies targeting key infrastructure areas for carbon reduction initiatives (e.g., data centers, transportation, waste management).
Article
AI for a Greener, Sustainable World
By Curt Hall
Posted November 10, 2021 | Sustainability | Leadership | Technology | Amplify
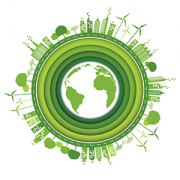
Don’t have a login?
Make one! It’s free and gives you access to all Cutter research.