There are myriad approaches that can help analytics projects deliver on their promises and potential. The articles in this issue of Cutter Business Technology Journal provide insights into some of these approaches. As you contemplate how to ensure that your organization derives value from your next analytics initiative, it is imperative to develop your own path forward, determining what tactics you feel will work best in your environment.
Article
Analytics Value Now! — Opening Statement
By Dave Cherry
Posted June 21, 2021 | Leadership | Technology | Amplify
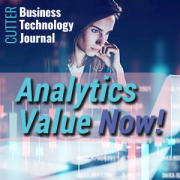
Don’t have a login?
Make one! It’s free and gives you access to all Cutter research.