Increasingly, government agencies, businesses, universities, and other organizations are working together to build AI systems that analyze geo-mapped and other location-based data. One such impressive new ML application uses Uber driver data to track and alleviate urban traffic congestion. It is designed to give urban transportation analysts and traffic engineers access to information about city traffic patterns in order to relieve bottlenecks, chokepoints and other problems.
Advisor
Big Data, ML, and Geo Mapping for Real-Time Traffic Monitoring and Analysis
By Curt Hall
Posted December 15, 2020 | Technology |
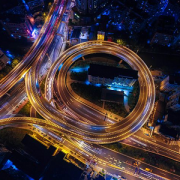
Don’t have a login?
Make one! It’s free and gives you access to all Cutter research.