The increasing realization that deep learning alone cannot be the solution to build robust, reliable artificial intelligence (AI) systems, coupled with the ever-increasing need to make use of heterogeneous data sources for decision making, has led to a recent resurgence of knowledge graphs (KGs). KGs are now playing a seminal role in the emergent field of neuro-symbolic AI, which aims to integrate domain knowledge into AI systems. By combining AI’s statistical/machine learning (ML) side with KGs, we get more effective, more explainable cognitive results and begin creating logic-based systems that get better with each application. In other words, we can build the next generation of AI models, ones that support better human-machine collaboration.
Article
Connecting the Dots with Knowledge Graphs — Opening Statement
Posted August 10, 2022 | Technology | Amplify
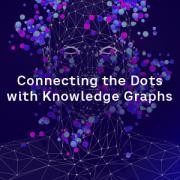
Don’t have a login?
Make one! It’s free and gives you access to all Cutter research.