This issue of CBTJ will help you understand that a data architecture should be much more than merely a technology roadmap. To be of any value to people in an organization, the architecture should guide the people in an organization to an understanding of how to organize for ever-changing information requirements.
Article
Data Architecture Is Really About People — Opening Statement
Posted January 17, 2020 | Leadership | Technology | Amplify
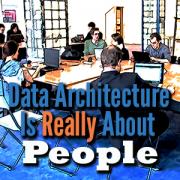
Don’t have a login?
Make one! It’s free and gives you access to all Cutter research.