The application of advanced technologies, including artificial intelligence (AI), are increasingly being used to detect wildfires before they grow out of control, predict a wildfire's path, and assess the risk and potential damage caused by wildfires. This Advisor explores the application of AI and machine learning technologies to mitigate the impact of wildfires.
Advisor
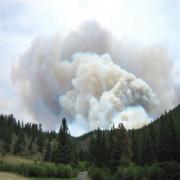
Don’t have a login?
Make one! It’s free and gives you access to all Cutter research.