Although still in development, products and services utilizing FHE are available. Organizations should consider investigating the technology now in order to prepare for when it starts to enter the mainstream. This Advisor examines some companies, products, and services utilizing FHE that can help you in your investigation.
Advisor
Fully Homomorphic Encryption — Vendors, Products, and Services
By Curt Hall
Posted April 6, 2021 | Technology |
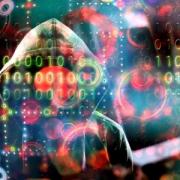
Don’t have a login?
Make one! It’s free and gives you access to all Cutter research.