Explainable AI (XAI) is the discipline of going deeper within the AI system, identifying the reasoning behind the recommendations, verifying the data, and making the algorithms and the results transparent. XAI attempts to make the analytical recommendations of a system understandable and justifiable — as much as possible. Such explainability reduces biases in AI-based decisions, supports legal compliance, and promotes ethical decisions.
Advisor
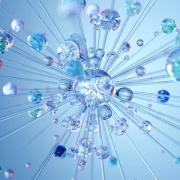
Don’t have a login?
Make one! It’s free and gives you access to all Cutter research.