Rohit Nishant and Thompson S.H. Teo discuss how to limit the negative impacts of artificial intelligence (AI) adoption by using concepts from both regenerative and doughnut economics. These two approaches seek to reconstruct economic systems so they operate within the sustainable operating limits of natural systems. Pointing out that AI adoption threatens to exceed sustainable boundaries by increasing aggregate demand for energy and new materials, Nishant and Teo put forth a 3Rs framework with which AI adopters can keep the impacts of AI within sustainable boundaries.
Article
Greening Data Management for AI
Posted May 23, 2022 | Sustainability | Technology | Amplify
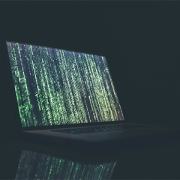
Don’t have a login?
Make one! It’s free and gives you access to all Cutter research.