Various AI technologies, including ML, predictive analytics, NLP, and image recognition, are helping to reshape procurement operations. These developments are most apparent in the emergence of AI-powered cloud procurement platforms. This Advisor provides some example use cases of how AI is now utilized to automate and optimize procurement activities.
Advisor
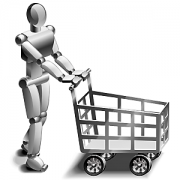
Don’t have a login?
Make one! It’s free and gives you access to all Cutter research.