In this Executive Update, we look at how product intelligence helps address challenges to achieving product leadership, as well as the general business improvements that improved product data and insight can drive.
Executive Update
Information Superiority from Product Leadership
Posted September 23, 2020 | Leadership | Technology |
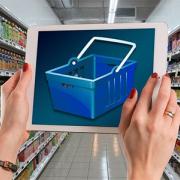
Don’t have a login?
Make one! It’s free and gives you access to all Cutter research.