While it is impossible to predict the future, the ability to adapt to what we already know is most likely to prompt a solution’s future pivot can make the difference between an elegantly evolving architecture and one that must be thrown away. In our experience, the patterns and techniques discussed in this Advisor are useful for keeping our digital and data architectures open to changes.
Advisor
3 Ways to Keep Your Options Open
Posted February 3, 2021 | Technology |
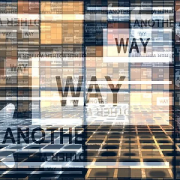
Don’t have a login?
Make one! It’s free and gives you access to all Cutter research.