Andy E. Williams looks at how human-centric functional modeling (a way to allow computers to solve general problems) could be used to create KGs capable of providing compete semantic models of systems, enabling us to transition to Industry 5.0. He defines Industry 5.0 as a world in which far greater integration is possible, including functional computing approaches like GCI. Although the emergence of GCI isn’t guaranteed (it could end up in a technology gravity well), it would bridge type 1 and type 2 reasoning and lead to a radical increase in our ability to solve every problem.
Article
Knowledge Graphs & General Collective Intelligence: Shifting to Industry 5.0
Posted August 10, 2022 | Technology | Amplify
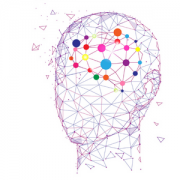
Don’t have a login?
Make one! It’s free and gives you access to all Cutter research.