Cigdem Z. Gurgur looks at KGs in the context of blockchain. The article begins with background information on how KGs have been used in advanced analytics and their role in helping AI developers. Gurgur then shows how blockchain’s immutability and verifiability offer designers a way to advance KGs to produce more reliable results. The blockchain/KG combination is an ideal one to build more explainable AI systems, she says. Finally, Gurgur explains how KG-enabled information systems can be used in industrial settings to enhance product development lifecycles, improve factory safety, and enhance information systems to the point where employees need less technical knowledge to perform their duties.
Article
Knowledge Graphs Meet Blockchain: Boosting Productivity in Industrial Products with Trustworthy & Explainable ML
Posted August 10, 2022 | Technology | Amplify
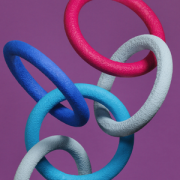
Don’t have a login?
Make one! It’s free and gives you access to all Cutter research.