As a fast-evolving area, AI presents innumerable opportunities and applications that we haven’t even imagined yet. This issue of Cutter Business Technology Journal discusses the current factors and considerations surrounding AI today and take a look at where trends might be heading in the future.
Article
Navigating the Prospects and Perils of AI — Opening Statement
Posted June 9, 2021 | Leadership | Technology | Amplify
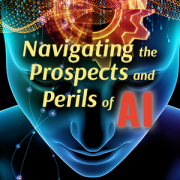
Don’t have a login?
Make one! It’s free and gives you access to all Cutter research.