BT & DTS EXECUTIVE UPDATE VOL. 20, NO. 6
Business on Autopilot
A picture is worth more than a thousand words. While we’ve been discussing how to capture the essence of the concept of information superiority, a YouTube video, “Tesla Autopilot Predicts Crash,” went viral and was quickly picked up by the media. At first glance, the clip is just another recording of a car accident captured by an onboard camera. What sets this one apart, however, is the fact that viewers can hear the autopilot alert and see the car reduce speed before the situation on the highway actually gets messy, protecting the lives and health of the passengers.
There are three important observations regarding what this smart machine — a Tesla with self-driving software — achieved. First, the car was able to perceive its environment autonomously and filter essential information from its sensors better than any human could. Second, using this ability, the car predicted the risk of a crash in front of a driver. And finally, the vehicle acted on this information by decelerating the car and avoiding an accident. When viewers see this video, they often react emotionally. YouTube comments range from “Wow! How is it even possible?” to “Another great reason to put that technology into all cars.” An undisclosed desire — “I want one!” — seems to underlie even the most constrained comments. This emotional reaction is not about sleek car design or its technical superiority measured in horsepower, speed, or acceleration. It is about machine intelligence and our expectations of a radical and largely positive transformation of the driver and passenger experience.
The ability to “observe, orient, decide, and act” (OODA) better than peers is the cornerstone of information superiority strategy that companies playing in the digital market strive to achieve. Does it mean that we are about to see all business models and business operations built around some kind of “business autopilot”? Will the process of strategy definition and monitoring resemble interaction with navigation systems or real-time strategy games? Arguably, the right questions might be “When?” and “In which kinds of businesses?” rather than “Is this going to happen?”
Certainly, as autonomous cars would not exist without the evolution of human drivers’ skills, step-by-step improvements in car automation, and traffic management infrastructure, information superiority needs to be well understood, implemented, and improved by people before we can responsibly pass business management to artificial intelligence (AI). Nevertheless, the succession of command from humans to machines is becoming a reality. It’s already happening in the financial industry with the advent of companies such as Aidyia, an AI investment management firm created by AI guru Ben Goertzel, which is basically an AI-based platform “packaged” in a legal construct. For any market, the emergence of AI-driven business models increases the importance of information superiority as the key enabler of competitive advantage in hypercompetitive markets that are permeated by technology.
Digital “Arms Race”
Hypercompetition — a business environment where barriers to entry are extremely low, where successful products can be easily imitated, and where key resources are abundant — makes competition a tough game. Today, substantial parts of business are a digital playground. Technology is commonly used to initiate and mediate relationships, capture and automate processes, and inject intelligence into everyday objects, spaces, and content streams. This heats the competition even further, accelerating the flow of value propositions, disrupting revenue streams, and increasing the convergence of industries.
The players in digital business are left with relatively few viable strategic options. The first is to become a customer experience (CX) leader in a well-selected category of CX-based services (e.g., online entertainment, electronic and mobile commerce, or travel assistance). Second, smart utility strategies enable and monetize an ecosystem of business partners providing value-added offerings to a portfolio of well-executed core capabilities (e.g., core banking services supporting fintech players or digital services provisioning and billing supporting “over the top” service providers). The third option, an ecosystem enabler, acts as a lean wholesaler of capabilities for an existing ecosystem (e.g., international logistics capabilities supporting pure e-commerce players or international VoIP termination for communication services providers). Finally, a business may seek ways to act smarter than its competitors through the following: achieving a better understanding of the environment (i.e., customers, partners, competitors, regulators), gaining better insight of its inner workings (i.e., assets, processes, capabilities), and honing a superior capability to turn information into effective actions.
The strategy that focuses on these goals is information superiority. We borrow this phrase from authors David S. Alberts, John Garstka, and Frederick P. Stein, who penned the seminal book Network Centric Warfare, which was funded by a US Department of Defense (DoD) research program and aimed at improving the DoD’s understanding of national security implications of the Information Age.
Businesses should execute strategies that are reasonably sustainable. This is extremely difficult in a hypercompetitive environment, especially where CX leadership is concerned. Sustainability of experience leadership requires the capability of continuous innovation of value proposition and its delivery. Ecosystem enablement and smart utility strategies offer better sustainability, but these strategies are complex and require huge economies of scale. They are best executed by market share leaders, which is a status few businesses can achieve. So the question remains: where does information superiority fit on the landscape of “digital strategic options”? (see Figure 1).
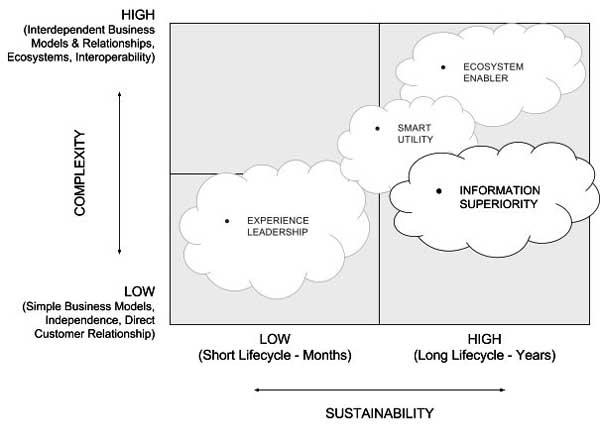
Figure 1 — Landscape of strategic options in the digital age.
Before answering that question, note that while other strategies can to some extent be seen as alternatives, information superiority is different; it should be implemented in combination with any of the other options, serving as a “booster” of competitive advantage. In this Executive Update, we propose possible scenarios for the implementation of information superiority strategy, which provides relatively high sustainability without introducing too much complexity.
The Cornerstone of Information Superiority
Adaptive Loop
First, we would like to define the concept of information superiority in a more precise way. For this purpose, we would like to use a reference model of an adaptive information/decision loop showing how information affects the ability to compete in a hypercompetitive environment: a business battlefield. The model we use here — the so-called OODA loop — comes from US Airforce Colonel John Boyd, who used it to present concepts of maneuver warfare (see Figure 2).1 Apart from being adopted into modern military thinking, the concepts of maneuverability and the OODA loop have also gained growing interest in business and IT communities, being referenced by industry analysts, scholars, and business literature authors.
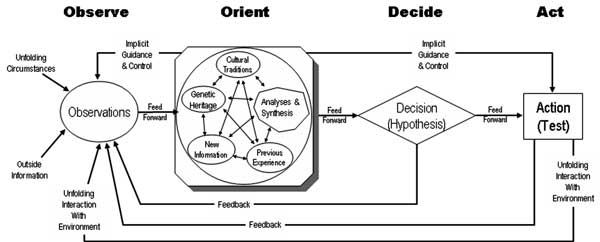
Figure 2 — Boyd’s OODA loop.
The flow of information between the OODA areas, along with actions specific for each of the areas, create a series of loops shaping the behavior of an entity in a competitive environment — be it a fighter pilot engaged in a dogfight or an organization in a highly competitive market. Such bold generalizations can raise suspicions. The fighter pilot, his plane, and his opponent represent a fairly monolithic, isolated system. On the other hand, organizations — with their external and internal relationships, within the context of their ecosystem — are complex, interdependent, and “fuzzy” in their behavior. One should understand, however, that the evolution of Boyd’s thinking extended a long way from his personal dogfighting experiences. Through the concept of air combat tactics, Boyd’s thorough study of conflicts, military doctrines, philosophy, and engineering evolved to the point where a mature, “distilled” OODA was successfully adopted as one of the cornerstones of modern maneuver warfare as practiced by elite troops.
The military origins of OODA and network-centric warfare may raise objections about the applicability of these concepts in business. After all, business is primarily about the relationship with the customer, not about busting competitors. While we are not militarists, we agree that successful implementation of experienced leadership strategy is a tough rivalry for customer attention and engagement. This is where you need to be able to spin fast cycles of activities that result in the following: understanding the customer, positioning and convincing them about your value proposition, and playing every advantage over substitutes to buy, use, and appreciate your offering. You need to turn every touchpoint in your customers’ journey into interaction that strengthens your relationship with them and does not leave room for your competitors to introduce doubt, disappointment, uncertainty, or fear about your offering. For this reason, we believe OODA offers valuable “lenses” when looking at information superiority in hypercompetitive business.
Boyd points to a number of critical factors influencing information superiority (which come from his writings and from the briefings as reported by his biographers and acolytes). Next, we present these critical factors, their applicability to business, and their subsequent consequences.
Must Go Faster
From the perspective of the OODA model, the key to winning a competitive game is executing the OODA loop faster than the opponent. Maneuver warfare gurus speak of the capability to operate “inside the opponent’s OODA cycle.” Such capability allows one to disrupt opponents with otherwise equal capabilities, by rendering their responses late and thus inadequate to the current “operational picture.” To increase your overall OODA speed, we suggest identifying business capabilities where speed is most relevant for your strategy and scrutinizing them for cycle time improvements through the OODA lenses (see Table 1).
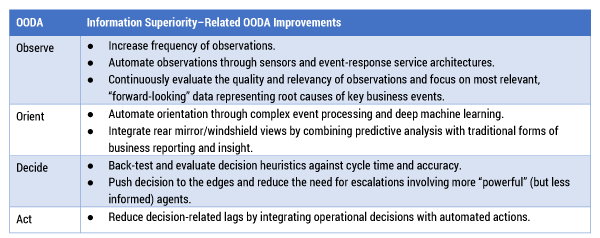
Table 1 — Sample OODA performance improvements actions.
Orientation: The Heart of Smartness
The heart of the OODA loop — and arguably the area where information superiority is born and maintained — is the orientation. In this phase, data is transformed to a meaningful picture of reality to enable purposeful actions to be taken in order to exploit opportunities or mitigate risks. The orientation phase exploits various decision heuristics and conceptual and analytical models used to evaluate information and identify events that should be appropriately acted on in the “decide, act” part of the OODA loop.
Models and data used for orientation have their expiration dates. As the environment changes, even the soundest, well-performing models eventually become obsolete. Competitors copy and improve successful ideas and products, customers lose interest in once exciting experiences, and startups exploit technology innovations to disrupt entire market fields, changing the baselines of competition. This decay affects the entire OODA loop’s efficiency: the need for new orientation models may require a different profile of observations and changed decision processes.
Explicit Models, Implicit Actions
The fastest loops are those that do not require explicit decision making involving human actors. Implicit guidance and control, or reflex-like behavior that can be achieved through automation, training, and distribution of the decision-making process, lead to the best competitive performance, as long as the orientation mechanism provides for relevant “reflexes.” To provide means for maintaining this relevancy, some form of “action evaluation” feedback should be implemented.
OODA-Oriented Architecture Principles
In this section, we outline our view of principles, practices, and architecture patterns that can be employed to achieve information superiority through systematically improving OODA performance. The following architectural principles define a solid foundation for OODA-oriented architecture.
Decentralized Operations
The separation of “thinking” from “doing” and escalations are major enemies of maneuverability. They introduce delays and errors, prohibiting quick, pragmatic decision making and agile responses to events on the “marketfield” (i.e., the business battlefield). Focusing on OODA performance implies, therefore, that business should be architected in a way that allows for substantial autonomy and decision-making authority of agents responsible for executing strategy and delivering results.
The most obvious approach to architecting decentralized operations would be to start with units representing areas of a product/customer matrix: line of business (LOB) units. Such units should have sufficient operational capabilities to take full, end-to-end responsibility for customer experience and business results (e.g., customer metrics, revenue, margin) in their respective marketfield. They can be seen as “containers” encapsulating essential capabilities (e.g., product lifecycle management, CRM, resource management), related operational and tactical business cycles (e.g., lead-to-order, order-to-cash, idea-to-service/product, problem-to-solution), and business cycles related to critical conversions in the customer journey for companies that implement CX leadership strategy.
This approach to business architecture may seem obvious, but it heavily affects the hierarchical organizations — their chain-of-command and functional silos (or should we say, dominions). As LOB units build capabilities essential to delivering and monetizing customer experience, questions may arise about the efficiency of such decentralized design. As a rule of thumb, if a function has a high impact on customer experience and requires product specialization and/or customer intimacy to be executed well, then centralization of this function will most likely derange OODA performance of the LOB unit. On the other hand, redundancy of routine operations, which are executed in a uniform way regardless of customer segment or product, is most likely just a waste of resources. The transformation toward decentralized operations is always company-specific. Table 2 presents sample results of transformation from functional silos toward an LOB-based organization in a major communications services provider.
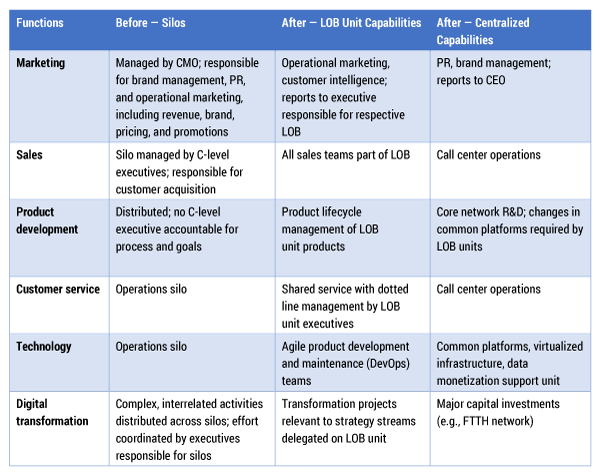
Table 2 — Example of business architecture transformation toward decentralized operations.
Collaborative Network
Decentralized operations transform organizations into an ecosystem of units that need to collaborate in many ways. A line of collaboration between autonomous LOBs must be maintained to enable a strategic consistency of business units across dimensions such as customer relationships, motivational systems, brand architecture, rules of engagement, or shareholders value.
No LOB unit is an island. It makes perfect sense to define them so that internal competition — the destructive force that ruins employee motivation and company image — is minimized. But in today’s convergent world, this may not be entirely possible. Collaboration usually makes much better tools for resolving related issues than escalation. LOB collaboration in processes such as product/market development allows for the creation of new business opportunities based on complex value propositions, combining the offering of different LOBs. For product/service delivery, a collaboration between LOB units provides for increased flexibility by sharing business-critical but underutilized resources,
Finally, as functional silos are replaced by end-to-end operations, LOB internal design becomes more complex than that required for function delivery. Therefore, it may be necessary to replace hierarchical chain of command with management teams that share responsibility for LOB performance while representing various facets of LOB operations. Excellent examples of the real-life application of the collaborative network concept can be found in the resources maintained by the “Beyond Budgeting” movement.
Adaptive Governance
For sizable OODA-oriented organizations, governance is a critical tool enabling distributed, collaborative operations. The most important aspects of such governance include the following responsibilities of C-level executives:
- Managing a reasonable set of “rules of engagement” for LOB units, which provides a clear mandate for frontline leadership along with transparent accountability framework for LOB management teams
- Strategy deployment (leading a collaborative process starting with “strategic intents” and elaborated with management of LOB units to form a roadmap of strategic initiatives and goals) and its continuous realignment with the evolution of the marketfield
- Maintenance of performance benchmarks (internal and external), which serve as a tool to assess the relative performance of LOB units and support an integrated view of business results and trends
From the OODA perspective, governance provides strategic-level orientation. This capability helps turn the understanding of company business (e.g., perception of market opportunities, strategic intentions and commitments, company competitive potential) into a system of strategy execution, reflected in the structure of its distributed operations network and processes that motivate, coordinate, monitor, and provide feedback.
As our understanding of the business and its environment evolves, the system needs to be realigned and improved. A flexibility of governance is required so that any need for incremental improvements (e.g., better customer experience, upgraded customer service standards, more efficient delivery) is observed and acted upon on the LOB unit level. But flexibility of any system of execution always has its limits. There are moments when the entire system needs to be changed. We have seen such market shifts in the past: traditional media versus digital publishers, mass marketing versus e-marketing, OTTs versus telecoms, fintech services versus banks, and traditional enterprise applications versus cloud service ecosystems. These shifts will inevitably become more frequent, and without adaptive governance, most businesses have little chance for long-term survival.
The leadership in an OODA-oriented organization is primarily achieved through influence, flexible and meaningful policies, leader development, and coaching. Executives make themselves available for LOB units as a resource supporting important activities. This is different from hierarchical command-and-
control structures, where leadership is more direct and often implemented through decision making, communication, and control. But the most important, and arguably most difficult act of leadership of
OODA-oriented organization executives, is the systematic reinvention of the business.
Shared Information Assets
Modern maneuver warfare doctrines advocate the need for a Common Operating Picture (COP) — a shared information resource supporting situational awareness of distributed actors undertaking and coordinating military operations. COP integrates data incoming from sources such as aerial surveillance systems, “shooters,” fixed and mobile sensors, autonomous vehicles, as well as command-and-control communication and intelligence systems. The data is accessed through a number of formats and views, tailored to the requirements and specific access channels (terminals) and users. It offers interpretation through different ontologies and levels of abstraction. Each user can be authorized to act as a supplier or consumer of the content for that resource. The principle of shared information assets (SIAs) is as important in business as it is in military operations. The categories of information that are or may be essential parts of such common business resource are:
- Internal and external performance benchmarks — the heart of management processes supporting collaborative network and adaptive governance principles
- Customer “digital trace” — context-rich data of customer interactions across all touchpoints used by LOB units that enable customer intelligence supporting business development or CX management
- Business intelligence (BI) services — supporting integrated customer and product analytics
- Management information services — supporting collaboration such as essential resource allocations and allocation plans, product roadmaps including LOB market objectives, and so on
OODA-Oriented Architecture Patterns
Apart from the principles listed earlier, we would like to mention a number of patterns that play an important role in OODA-oriented architecture.
Smart Business Cycles
A business cycle is a sequence of actions that represents a complete path of organization response to a business event. In OODA-oriented architecture, we capture the dynamics for LOB units by describing it as a collection of business cycles, supporting business capabilities, and IT assets (see Figure 3).
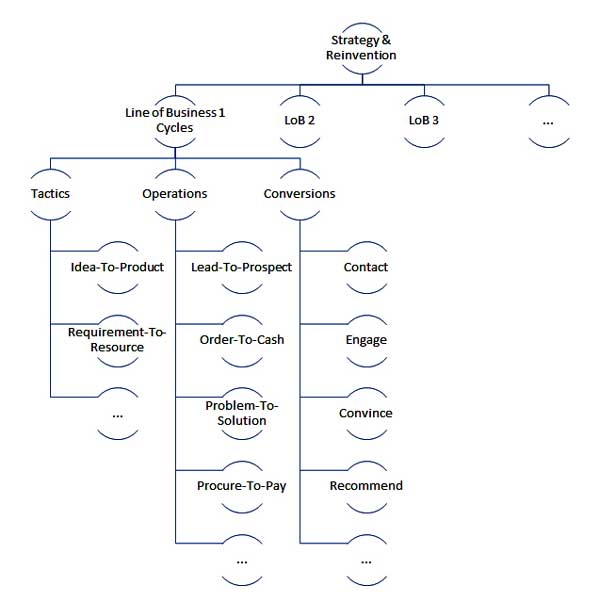
Figure 3 — LOB units as collections of business cycles.
A business cycle can be further defined as a specific case of OODA loop that opens up opportunities for information superiority–related improvements. The generic pattern for operational business is presented in Figure 4.
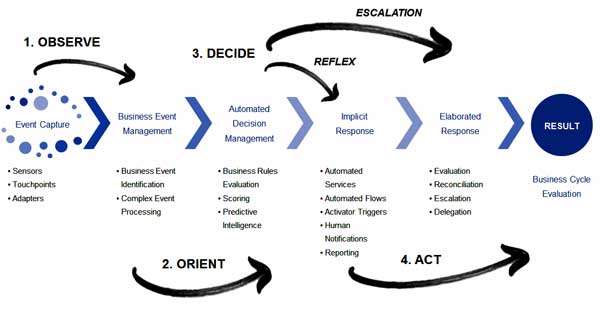
Figure 4 — A generic pattern for smart business cycles.
The data acquired from different sources (e.g., business website clickstream, transactions executed by business applications, temperature reading from a device sensor) is recorded as source-specific events. The data needs to be interpreted so that we can identify its business meaning (if any). For example, temperature readings can be stable, requiring no action, or they may signal a potential failure that calls for a preventive maintenance action. Moreover, a specific pattern of business events (e.g., a series of credit card payments at a specific location or a customer order placement resulting in product stock levels dropping below a limit) may trigger a follow-on context-based event, such as potential credit card fraud or stock replenishment notification.
Once the events have been identified and analyzed, rule sets specific for a given LOB unit and business cycle are evaluated for the most appropriate response to an event. It can trigger an automated service or an instance of automated business process that delivers the appropriate response, such as the addition of a stock replenishment task for a mobile robot in a product distribution center. Such a fully automated business cycle can be seen as a reflex — an activity that is executed without any need for human intervention.
In most real-life scenarios, the event-response activities might involve human intervention at some point. Personnel responsible for order completion in an e-commerce distribution hub may need to agree with a customer on a replacement product, a financial advisor may need to review complex investment options with her client, or an autonomous vehicle transporting goods is involved in an accident that needs to be investigated. Response for the initial event is not straightforward and needs to be elaborated by human actors through escalations to decision makers with more power or consultations with sources of expert knowledge sources (automated or human), or they may require a manual reconciliation for which automated procedures are not available.
This generic pattern can be adopted for a specific business cycle to serve as both an analytical and design tool. As an analytical tool, it helps to better understand how the existing business and IT architecture supports information superiority, discovering potential design gaps, limitations, and improvement opportunities along with what refactoring actions can result in better OODA performance for a given business cycle. Using the pattern as a design tool seems pretty straightforward as long as the task is well constrained, such as “Design the IT architecture supporting information superiority through high OODA performance for a specific business cycle.” Figure 5 provides some ideas of how technology can be applied across the business cycle to make it genuinely smart and efficient.
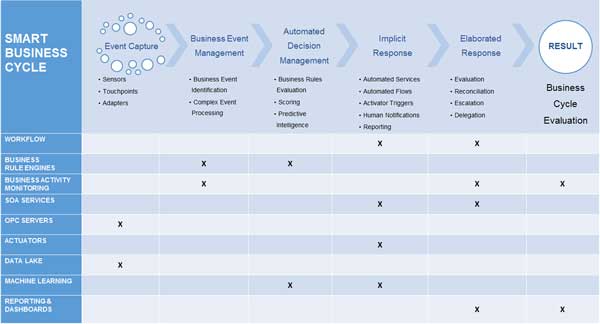
Figure 5 — Supporting a smart business cycle by IT assets and solutions.
Creating a general vision of OODA-oriented architecture for an enterprise is a more substantial effort that requires a pragmatic process, such as the one suggested in a previous Cutter Executive Report. To deliver the results encompassing essential business design aspects from the perspective of information superiority, the following artifacts need to be produced:
- The architecture of distributed operations (i.e., LOB units and supporting shared-service operations)
- For each LOB unit, their mission, constituting portfolio of products, collection of business cycles, key resources, IT assets (rule sets, services, applications), and corresponding essential business capabilities
- The adaptive governance framework essentials (i.e., roles, responsibilities, motivations, benchmark structure, and rules of collaboration between LOB units)
- The ontologies (conceptual models) that form SIAs and the platforms that support them; a data/service governance model for SIAs
Data Lakes and Ontologies
For decades, BI/data warehousing best practices assumed that analytical processes are employed to process information that is acquired, integrated, cleaned, enriched, and controlled within the confines of the organization. And the analytical models employed in most BI implementations have been fairly simple and relatively stable, as they reflected business scorecards, associated reporting, and variance analysis. Recent years show that these assumptions are no longer sufficient. Apart from “tried and true” data architecture patterns (i.e., data warehouses, data marts, ETL), there is a growing need to access in a manageable and systematic way data outside the span of control of a given organization. Many businesses realize that they will never be able to compete with leading search platforms, social networks, or ubiquitous digital communications platforms as far as the reach and richness of data is concerned. Moreover, the growth of the Internet of Things will increase the flow of data from sensors, devices, and related services.
What is important is the capability to understand the meaning of the data — both within its original context and format and within the conceptual model of a given LOB. We need to be able to map the original ontology — understanding events from the original data stream and their attributes, such as control signal and video transmission from a robot employed to assist customers in a shopping mall — to our business ontology: a potential customer requests information about a shop offering a given product category or a child in distress and possibly lost, for example. Even simpler cases, such as using programmatic marketing, require the mapping of customer profiles offered by a vendor (their ontology of Internet users) to profiles meaningful from the point of view of product targeting.The solution is to use the external data as if it is part of the internal data architecture. This can be achieved either by using programmatic approaches where the owners of the data offer services portfolios to access and process information and/or capture data streams, such as tweets, video/audio streams, and smart device communication, and store them for further processing in a native format with minimum processing. Such structures, often called “data lakes,” can be supported by several vendors and open source platforms (see Figure 6).
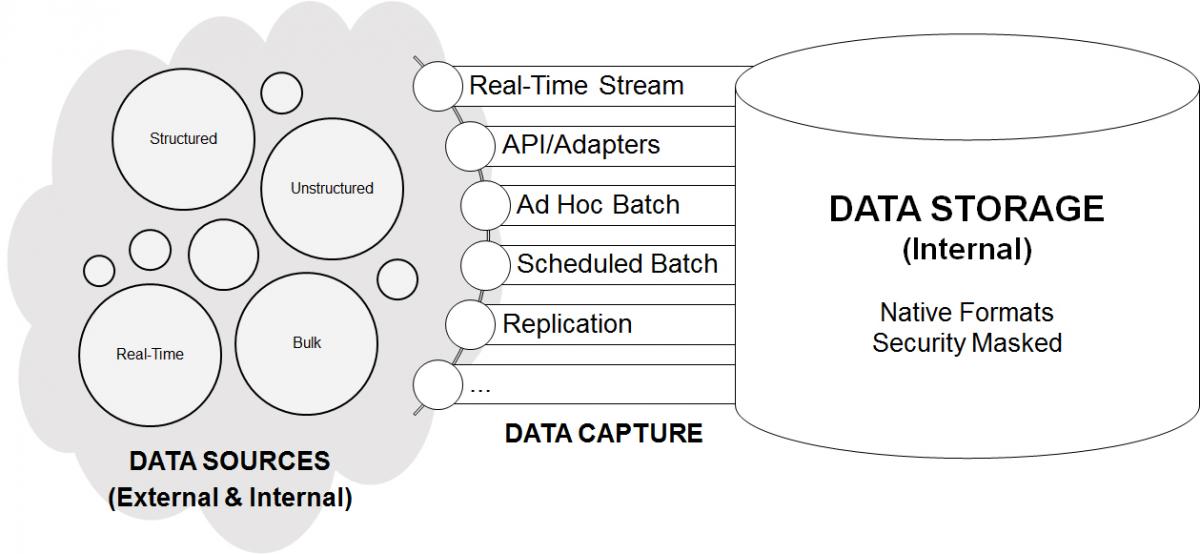
Figure 6 — Data lake architecture pattern.
Model Factories
With the abundance of information, the big data discipline is ultimately the discipline of asking the right questions. If we understand and exploit some important pattern of customer behavior, we can address the right people with the right message and at the right circumstances, and convert such business moments to a profitable exchange of value. The capability to systematically discover such patterns can translate directly to information superiority.
A model factory is a process and technology environment that discovers patterns and transforms them into analytical models that can be deployed in automated decision processes in areas such as recommendation engines, dynamic pricing solutions, fraud detection, targeting of promotional activities, optimization of logistics, or advanced automated interactions. A model is researched, developed, and tested in an analytical sandbox using data mining, specialized languages, advanced analytics, and visualization tools and methods (see Figure 7). Once accepted, it is implemented and deployed in a production environment. As OODA implies, the quality of any such model degrades over time because the business environment constantly changes. The model should be further monitored to ensure that it is still relevant and more effective than any other candidate model.
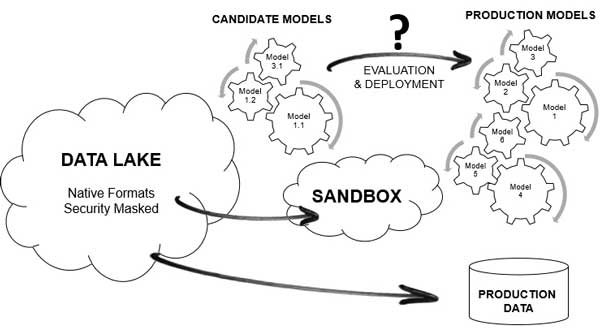
Figure 7 — Model factory architecture pattern.
In this approach, the predictive power of deployed models is constantly monitored; if there is a signal that they start to degrade, they are automatically rebuilt. In this way, the OODA loop is closed, allowing the organization to observe a continuous process of improvement. Modeling factories usually use a fully automated approach to model building and monitoring, as in typical scenarios, hundreds to thousands of models are used in a production environment.
The technology support for “orientation management” is tricky. First, it requires models — the lenses we use to interpret reality — to be explicit; otherwise, the task of evaluating a model or comparing the models becomes very difficult or even impossible. This is not something we are very good at when you look at the way most IT architectures are shaped. In many cases of real-world organizations, the automated business rules, reporting systems, data architecture, and KPI systems implement implicitly our understanding of the business and its environment. Flexibility of these solution is enforced by generalization of data or service architectures (e.g., the practice of building data warehouses on the foundation of normalized data models, supported by metadata management).
We assert that this approach will become insufficient to handle quickly changing, volatile, analytical models (e.g., fraud detection rule sets or targeting rules for marketing campaigns) or extensive usage of unstructured external data sets. For this reason, model factory patterns emerge as a pragmatic solution to this challenge.
Orientation (and orientation management) are inherently human, unstructured processes. In most organizations, information created from data by reporting systems, ad hoc inquiries, spreadsheets, and so on, are not interpreted as hard “traffic signs” but merely suggestions and hints confronted with human conceptual models and ontologies. These may extend our orientation process far beyond what we might call a “Vulcan approach” — rational inference from the provided data. As confirmed by psychological studies, our decisions employ gut feeling, emotions (How will I feel about it?), or moral judgement (Is it ethical?) to economic evaluation (Will it be profitable and efficient?). Moreover, learning and unlearning — or creating and destroying our conceptual models — is affected by the “psychology of change” that introduces friction and lag in the OODA cycle. The emerging disciplines that can enable us to reduce this kind of friction and lags, that have just hit the point of effective commercialization, are deep machine learning (ML) and advanced statistical analytics.
The Tesla accident, the 2016 defeat of Go World Champion Lee Sedol by AlphaGo, and subsequent triumphs of the platform over dozens of premier Go masters prove that orientation can already be automated in a way few might have expected a couple of years ago. The price to be paid is that while we control the architecture of ML solutions (e.g., the number and role of neurons and neuron layers and their internal “wiring”) and manage the learning process to large extent, we are often unable to understand specific results delivered by the system. They appear as black boxes even to their makers. Nevertheless, it seems inevitable that this price will be willingly paid by those looking for information superiority.
Demis Hassabis, the creator of DeepMind, of which AlphaGo is a spinoff, aims at “solving general artificial intelligence, and then solving everything else.” This implies that smart machines are going to have orientation capabilities, making human participation obsolete. Until (and if) this happens, we must understand that the human mind is still required to evaluate and improve the architecture of deep ML systems, and model factories are an important tactical solution to this problem. But all these innovations will be ineffective unless organizations adopt a culture of respecting evidence — measurable facts — as the essential driver of business and management decisions on every level.
Information Superiority Culture and Skills
Evidence-Based Decision-Making Culture
“Build it and they will come.” This approach may have worked for some visionaries determined to pursue their dreams against all odds. In the evolution of digital business, there is often room for a “technology push” strategy. When a technology innovation becomes ripe for addressing some obvious need, users adopt it naturally and effectively.
We strongly discourage adopting such an approach for sophisticated information superiority investments. From our experience, only the “demand pull” works in practice. Before implementing analytical model factories or advanced AI solutions, your line managers must be convinced that they want to adopt evidence-based decision making. Whether in marketing campaign targeting, investment management, customer claim evaluation, or product pricing, decisions can be based either on a “gut feeling,” anecdotal evidence, stiff rules, or fact-based insights. For some organizations, the former style may appear good enough, especially when an organization lacks data to enable evidence-based decision making. But evidence-based decision making, supported by quality information, will usually outperform less disciplined styles when the business environment becomes more turbulent and customers more demanding. The clue is to develop the business practices, tools, and data (or, as we call it, “digital capital”) evolutionarily, so that organizations continuously improve their total capability to observe, orient, decide, and act.
Once they are developed, the appetite for facts, insights, and predictions can be satisfied and new areas of applications emerge naturally. Additionally, there is a natural low level of support when the implementation of analytical solutions requires the processes in the organization to change. In our experience, it is simplest to start with small and relatively simple analytical projects so that mid-level managers can gain confidence that the benefits are worth the effort.
Efficient Information Management Processes
If we were to point to a single reason why data science projects fail even in organizations committed to evidence-based management principles, it is the quality of the data. The often-used saying “garbage in, garbage out” applies extremely well to decision-making processes. Here we understand data quality in a broad sense. The key concept is that people in the organization need to trust the results of the analysis and be confident they are credible, actionable, and safe to use in automated processes. Technical solutions can be built, but there is no way evidence-based management can be established and developed in the absence of the “evidences” that might support decisions.
In particular, we often find that before analytical models are built, an organization must change the data collection (observation) practices or even change its business processes in order to be able to perform meaningful analysis. An example might be the A/B testing of website design. In order to be able to optimize content presented to a potential customer, you first have to create a capability to perform marketing experiments on the website. The creation of such an experimentation sandbox for more complex activities, such as fraud management rules, stock replenishment strategies in distribution business, or routing and obstacle avoidance for an autonomous logistics robot, requires an even more sophisticated sandbox environment, even if the process and tools for model design and evaluation remain similar.
Data Science Toolbox
Models must be built by data analysis experts. We believe that a key principle to make them effective is to give them the freedom to shape their tools and methods. To unleash their creativity, experts should not become overly constrained by corporate rules and regulations. In the past, the usual caveat was that sophisticated data analysis leads to significant software and hardware expenses. Fortunately, this has changed. On one hand, most of the tools a data scientist wants to use are available as open source. This does not mean that organizations do not need any budget to utilize them, but their license and maintenance cost footprint is significantly lower than in proprietary or legacy solutions. The other part of the costs is hardware. However, computing power can be bought really cheaply as big players such as Amazon, Microsoft, and Google wrestle to maximize their share in this rapidly growing market.
Conclusion
The information superiority capabilities described in this Update are important for building a competitive advantage. While we believe that information superiority provides more sustainable advantage than CX leadership, there is no guarantee of decades-lasting advantage in a hypercompetitive marketfield. In the long run, your competitors will also start to make evidence-based decisions, improve their data governance, or build modeling factories. Data science skills will commoditize. Perhaps the only asset that can, if properly managed, bring a long-lasting competitive edge is ownership or privileged access to data and logic, which can be monetized by OODA performance or by commercial, programmatic (API-based) access; in other words, digital capital.
Time is crucial for information superiority, and not just because it is a critical factor in OODA performance. Think of the Tesla example. In theory, any company has access to AI technology, allowing it to build a self-driving car. The advantage of Tesla in this field is that it has invested in the collection of data that allowed for training these algorithms. This information is scarce on the market, exclusive, and actionable. Additionally, it is expensive and time-consuming to collect information of similar quality, and Tesla’s knowledgebase expands every day, so you are chasing a constantly moving target. Therefore, if information superiority seems to be the name of the game in your business, do not lose time. Start early and move quickly.
1 Boyd disseminated his ideas in the 1970s and 1980s through numerous briefings given to members of the defense community. The content of these briefings has changed over time, echoing the evolution in Boyd’s thinking. OODA was introduced in briefings named “Patterns of Conflicts” and “The Discourse on Winning and Losing,” later becoming one of the focal points for a series of briefings given the common title “The Essence of Winning and Losing.”