Northwestern Medicine has developed a very innovative NLP application — integrated within the hospital EHR system — designed to solve a practical and pressing healthcare problem: missed follow-ups associated with incidental findings from diagnostic imaging. This Advisor explores the significance of this innovative artificial intelligence application.
Advisor
Northwestern Medicine’s RMS: Improving Patient Care with AI Imaging Innovation
By Curt Hall
Posted May 11, 2022 | Technology |
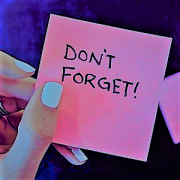
Don’t have a login?
Make one! It’s free and gives you access to all Cutter research.