This issue of Cutter Business Technology Journal explores various angles to big data with a focus on the trends in predictive analytics, ML, IoT, and the cloud.
Article
Trends in Big Data Technologies and Analytics — Opening Statement
Posted December 11, 2017 | Leadership | Technology | Amplify
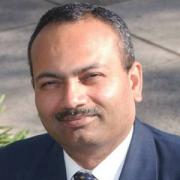
Don’t have a login?
Make one! It’s free and gives you access to all Cutter research.