CUTTER IT JOURNAL VOL. 29, NO. 6
Data is important, but I prefer facts.
— Taiichi Ohno, originator of the Toyota Production System
In the closing chapter of The Innovators, the story of the emergence of the computer age, Walter Isaacson explores the history of computing and speculates on its future. He explains that cognitive computing — once called “artificial intelligence,” the notion that computers will be able to “think” — has perpetually “remained a mirage, always about 20 years away.”
Suddenly we are witnessing the convergence of many advances — from self-driving vehicles to new medical diagnostic and delivery methods — and the fruition of these breakthroughs may deeply impact us all in unforeseeable ways. While there are many differing views, and even fears, about big data and cognitive computing, there is also tremendous opportunity. As Isaacson concludes, “New platforms, services, and social networks are increasingly enabling fresh opportunities for individual imagination and collaborative creativity. This innovation will come from people who are able to link beauty to engineering ... humanity to technology.”
We must make wise choices about how we invest in and use these emerging technologies. So the premise of this article is this: how do we ensure that we are getting the most from big data, cognitive computing, and whatever lies beyond, to improve the probability of making the right decisions, in the right context, and for the right reasons? We believe that lessons learned in over five decades of Lean Thinking can help guide us forward in this journey, and we will use examples from the financial services industry to illustrate them.
The Essence of an Adaptive Learning Organization
From the beginning, there were two branches of artificial intelligence: one that seeks to replace human cognition and one that seeks to augment and complement it, to make it more effective. Of these two paths, Isaacson observes, human-computer symbiosis has been more successful. “The goal is not to replicate human brains,” says John Kelly, the director of IBM Research. “Rather, in the era of cognitive systems, humans and machines will collaborate to product better results, each bringing their own superior skills to the partnership.”
Whether computers will ever really “think” may forever remain a philosophical and theological debate. Nevertheless, we can expect that computing will increasingly augment and even replace humans in many circumstances, just as robotics and other technology advances have done in recent memory. So what is the right approach to optimize human-computer interaction?
In 1983, just as the notion of a personal computer was becoming popular, Eiji Toyoda, the Toyota chairman who supported Taiichi Ohno in the creation of the Toyota Production System (the origin of Lean), made this observation:
Society has reached the point where one can push a button and be immediately deluged with technical and managerial information. This is all very convenient, of course, but if one is not careful, there is a danger of losing the ability to think. We must remember that in the end it is the individual human being who must solve the problems.
A Lean organization encourages every individual to actively seek out problems (rather than avoid or deny them), because problems are the catalyst for continuous improvement and innovation. Lean practice is founded on the scientific method of problem solving; information enables the perpetual feedback loop of continuous improvement and innovation toward the creation of value for the customer. While Lean practice emphasizes data-driven decision making, it must be done with the proper understanding and context, hence the importance of gemba — the idea that one must go to the source, to where the work is done, in order to fully understand the situation.
One interpretation of gemba is context and situational awareness through firsthand observation. A Lean management system encourages those closest to the customer, those who best understand the work, to own their processes, solve their problems, and make their own improvements and innovations, guided by the strategic priorities of the overall organization.
To achieve this new way of thinking and acting, managers must step away from their PowerPoints and spreadsheets, leave their offices and meeting rooms, and actively observe the situation in person. They must overcome the tendency to think they know the answers and learn to rely on the understanding of those who deal with the problems on a daily basis. Their role changes from command and control to coaching, providing support and encouragement to help others overcome obstacles and develop insights into cause and effect, so they can continuously and sustainably improve their work.
Not only does this result in better processes and better problem-solving skills, it nurtures future leaders. Lean requires a whole new style of leadership and management. As Jim Womack (coauthor of Lean Thinking) insists, “The Lean manager realizes that no manager at a higher level can or should solve a problem at a lower level; problems can only be solved where they live, by those living with them.”
Being closer to the problem is not just a matter of cutting through vertical hierarchy; it requires a contextual shift to the horizontal flow of value. Value streams begin with a need expressed by a customer and conclude with delivery and satisfaction, which creates a complete end-to-end perspective. A Lean approach to problem solving must thus begin with a deep understanding of the organizational architecture — breaking down functional silos and their counterproductive attitudes, measures, and incentives in order to create what is often called a “line-of-sight view” to the customer needs and experience.
Consider the nature of value streams in today’s marketplace. Teams are often geographically distributed, and customers may be globally dispersed and very diverse in their characteristics and consumption patterns. While “going to gemba” to talk and learn directly with customers will always be valuable in gaining new insights, these face-to-face conversations will be with a very small, often non-random sample of a larger, diverse population and will not provide the breadth of insight that a larger sampling can provide.
Big data analytics can play a vital role in directly engaging individuals and teams at all levels within the enterprise with the virtual voice of the customer, creating an instantaneous line-of-sight view. This continuous feedback and learning provides an efficient and rapid way to design and conduct experiments of any scale to explore customer preference and actions, enabling individuals and teams to make decisions that continuously align with and inform enterprise strategy.
Seeing the Whole
People often say that “politics” or “culture” gets in the way of effective decision making. What this usually indicates, in Lean terms, is that individual functions attempt to locally optimize the segment of the value stream for which they have responsibility and by which they are measured and rewarded. This behavior leads to suboptimization, where segments of the value stream may compete with each other, often pushing the problems and waste into another function’s area, like pieces on a game board, while not adding value (speed, quality, cost, safety, experience) to the end customer.
While each function typically has abundant data at the start of an improvement effort, this data is usually very messy — it is strongly influenced and filtered by how each value stream segment is measured and rewarded, so collectively the data does not represent the information essential to improve the flow of the overall process. Once data, evidence, artifacts, and anecdotes across the entire end-to-end value stream are gathered in one place, and as the team fully engages in value stream mapping and analysis, together they come to realize that data from each segment reflects a gathering of suboptimal and often counterproductive points of view. At this moment, someone on the team is often heard to exclaim, “I have been working here X years, and this is the first time I truly understand why this process just doesn’t work!”
Simply normalizing the composite data does not correct the distorted perspective on the overall flow. The team must together map and analyze the value stream, determining what are the key drivers for improving the end-to-end flow that transcend individual interests, metrics, and incentives. The inherent unreliability of technically normalized data (which creates the appearance of coherence) poses a significant challenge for effective data-driven decision making. Lean thinkers have learned that when a process is not well understood from end to end, they should not begin with a data analysis deep-dive. First everyone must visualize the overall flow and context of the process, transforming a disparate gathering of stakeholders into a purpose-driven team. With that understanding, the team can together identify and collect the relevant data and prioritize which problems must be solved to optimize that collective, overarching purpose. Then they can design experiments accordingly to test the impact of changes on the overall outcomes.
The team must look at both performance to the overarching goal and the contribution of each value stream segment toward that goal to optimize value and performance for the customer. If this collective “clarity of purpose” isn’t deliberately nurtured, suboptimized improvement efforts — while creating the appearance of progress — can actually drive the value stream and its participants deeper into dysfunction and despair. Misguided big data efforts can do that as well, despite best intentions of everyone involved.
What Makes the Whole?
What might be streamlined and automated today may be entirely replaced tomorrow by innovative, disruptive human creativity. As the strategist Arie de Geus observes, “The ability to learn faster than your competitors may be the only sustainable competitive advantage.” This is the essence of Lean Thinking and how it can transform the behavior and culture of an enterprise, preparing it to compete in a highly disruptive future.
Machine learning can help people gain new insights and make informed decisions, but people can’t always turn the running of a process entirely over to machines, especially within a complex and dynamic system. While a machine may surface hidden signatures that no human would think to look for, we strongly believe that humans must be able to see that result and, at a minimum, hypothesize the mechanics that allow for a relationship between those new Xs and the Ys. This requires special insight and creativity.
If the analytics engine is a black box to the people responsible for the work, imposed on them by experts, it can become a blunt instrument of management command and control. Automated monitoring and sophisticated analytics can help the team to monitor the state of a process, and instantly sense and respond to deviations, but the team must understand their process to understand the meaning of the deviations so they can solve the right problems and make the right decisions to achieve the desired outcomes.
Big data has already demonstrated many successes, and experts assert that cognitive computing systems can actually make the context behind decision making “computable,” acting as a proxy for human intuition. It is that convergence — human creativity supported by relevant information — that offers the greatest potential.
From a Lean perspective, then, how and when can we make context computable in order to help those who are closest to the problem? To address this question, let’s briefly examine the fundamental problem-solving journey embodied in Lean Thinking. In his article “Purpose, Process, People,” Jim Womack observes:
... business purpose always has these two aspects — what you need to do better to satisfy your customers and what you need to do better to survive and prosper as a business. Then, with a simple statement of business purpose in hand, it’s time to assess the process that provides the value the customer is seeking. Brilliant processes addressing business purpose don’t just happen. They are created by teams led by some responsible person. And they are operated on a continuing basis by larger teams led by value stream managers.
The further elaboration of Womack’s Purpose, Process, and People in Figure 11 illustrates the inner feedback looping and learning of continuous improvement, where the people responsible for the process are able to guide its improvement. When an organization is able to incorporate this mindset into their management systems, behavior, and culture, we can say they have become an adaptive learning organization.
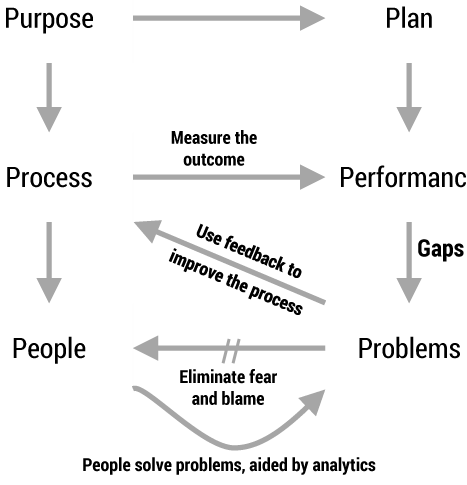
Figure 1 — The Six P Model.
A common challenge with traditional management systems, however, is that they attempt to control a complex, dynamic process from the top down and outside in, focusing primarily on outcomes. Trying to reward and punish those doing the work so as to achieve the desired outcomes can lead to tampering and gaming, where individuals and teams hit their quantitative targets but cause negative consequences in other parts of the organization. W. Edwards Deming argued for constancy of purpose and the abandonment of quantitative quotas, numerical goals, and the popular Management by Objectives approach. In their place, he emphasized skilled leadership.
Deeper understanding of the in-process measures can help teams understand the true drivers of performance, regardless of where the responsibilities and accountabilities reside, so they can continuously fine-tune to produce the desired outcomes, especially in a complex and dynamic system. Paradoxically, to achieve desired outcomes, managers must pay more attention to the process and less to those outcomes — it is the internal process drivers that we must analyze, with a keen eye for cause and effect.
Case Studies: Problem First or Data First?
Let’s now apply our Lean Thinking insights to better understand two distinct approaches to using big data: problem first and data first, also called supervised and unsupervised machine learning. The first requires a hypothesis in order to utilize the data, while the second generates hypotheses from raw data. They are both useful for continuous improvement and innovation, but they require a different mindset from the practitioners.
Problem First
The problem first approach requires that we choose a problem, establish why it is important (purpose), then develop hypotheses, which are tested and result in learning (from either a positive or negative test) with the entire hypothesis-testing-learning cycle repeating iteratively as long as resources, demand, and prioritization against other challenges allow.
Hypothesis testing itself requires well-selected measurement points and good, stable data, which may be lacking. The underlying process problem being investigated is usually caused by variation, which contributes to challenges in effective data collection and analysis, especially when voluminous data is gathered through the machine learning process. The team must often take an initial step that focuses on measurement and data collection proofs-of-concept. Often a data cleansing effort is also needed to ensure the signal is clear (noise is minimized). The data itself, and the team that owns the process that creates/utilizes it, must essentially go through a mini-lifecycle to establish a baseline before the data can be used to guide the team toward continuous improvement.2
In the financial services industry, there are many specific challenges regarding timely deliveries of daily data needed to enable customer market activities. One very large financial services firm embarked on an effort to improve the delivery of a daily market calculation through enhanced automation and increased Straight Through Processing (STP), measured by how many (or few) times the calculation train stopped for human intervention. There was a strong perception within the team, and by management, that most late deliveries were caused by technology incidents. While the technologies were complex and challenging to integrate, the only measurements taken that could identify bottlenecks were post-mortem debriefs using subjective assessments — no real data was captured for evaluation of the process.
After just a few months of measurement, visualization, evaluation, modeling, and reporting, things started becoming clear to the process owner. First, as the model revealed the most sensitive process steps, it was apparent that technology was not the primary cause of missed deliveries, as some had originally asserted. Second, there was a specific technology bottleneck that merited analysis. Due to the vast complexity of this single step, the team applied supervised machine learning, and the results were surprising.
When a single, unexpected transaction was revealed to create an excessive processing bottleneck, the team investigated the predecessor steps and rationale for that transaction. They discovered that there was a misunderstanding among the operations staff about how to best gauge technology process status — was the process complete or not? Frequent inquiry and process restarts were causing vastly increased system utilization, unnecessarily slowing down everyone’s calculations. This discovery helped the team develop a simple, fast, and noninvasive way to determine process status, which the users quickly adopted, increasing the available time between task completion and due date by 40%. This change led to improved delivery reliability for the customers and is expected to reduce operating expenses due to reduced errors, failure demand, and the resulting rework.
In this case, the team identified a problem, and through rigorous understanding of the process and collection and examination of the data that supported the process, they realized that their unquestioned assumptions were incorrect. This helped them to improve the process and the outcomes.
Data First
The data first approach is very different in nature. Computers are ideal for spotting hidden patterns and relationships that humans can’t see. But comprehending the relationships, in order to produce desired outcomes, requires context and situational understanding.
Another financial services case demonstrates the value of using computing power to reduce measurement intervals to provide actionable data. One value stream being measured was already achieving a high STP percentage, but the delivery against the customer satisfaction specification was still lagging. The technology team had a strong sense of where the problems were, but the team members were reacting to stale data that lacked client specificity in an area where much of the technology in each value stream is client-specific. While there were daily, even hourly, incidents that the team was responding to in a reactive mode, the overall performance of the value stream was only evaluated on a monthly basis due to the challenges associated with collecting, managing, and reporting on aggregated data for a continuous flow process servicing so many clients.
Here the challenge was to first solve the data and measurement problem. The team introduced standardization of measurements and automation of the data collection and reporting activities, reducing the monthly reporting lag from 20 days to five, and creating a daily report for the technology team, who consumed this information during problem-solving portions of their daily stand-up meetings. In parallel, a model was developed using data science to understand the process steps that were consuming the most time.
With their new ability to see emergent patterns, application developers were able to spot — and in some cases even anticipate — important events. By focusing on certain client sets and their specific technology architecture, they were able to quickly respond to deviations, thus establishing a clear connection between cause and effect, which helped them to drive out unnecessary variation. The measurement team also asked the technology team for more specificity in the incident ticket details to allow for better correlation between the user experience of process performance and the modeled behavior. This, along with technology process changes made by the subject matter experts, for example, reduced the delivery failure rate by 65% in one key client area alone.
An additional example of data first learning by this same team is a recent proof of concept using unsupervised machine learning to predict the extent of the client impact based on attributes known at the start of an incident. This is enabling real-time decision making by technology support staff as to where to focus their attention on rapid resolution of the more significant problems, and it may ultimately lead to preventative, and perhaps even predictive, countermeasures.
Purpose and People First
As we have shown with these business process improvement examples, both problem first and data first approaches can help teams sense and respond to emergent problems and opportunities, understand complex causal relationships, and continuously improve and adapt to changing conditions.
One of the most interesting aspects of big data, though perhaps disconcerting to some, is that it is not always necessary to understand causality. Insights can be gained by simply observing correlations gleaned from massive quantities of raw data gathered from diverse sources within large, complex systems, and useful patterns of behavior may appear even when causality is not understood.
An insight is useful, however, only if we can do something with it. Absent context and meaning, knowledge is simply interesting but not useful. In our view, we cannot reliably compute context and meaning when purpose isn’t clear. Human insight is necessary to frame a situation properly, interpret the analysis, and choose how to move forward with the design of experiments and adoption of improvement and innovation ideas.
To this end, pioneering data science professors at Harvard University propose a simple five-step data science process:
- Ask a question
- Get the data
- Explore the data
- Model the data
- Communicate the data
“While computers are getting better and better at doing steps 2, 3, and 4,” says Mark Basalla,3 lead data scientist at USAA, a financial services company, “only humans can ask the right questions in step 1 and tell the right story in step 5 in order to enable the right decisions to be made. Lean Thinking is an ideal way to help people do this consistently and effectively, through a variety of simple but effective problem-solving techniques and behaviors.”
In our experience with Lean transformation of large organizations, working with large cross-functional teams of human beings in complex and ambiguous circumstances, the question of purpose is often nuanced, involving the values and prevailing culture of the organization. A deliberate inquiry into purpose never fails to unlock deeper conversations, and it often leads to new understanding, creating energized, purpose-driven teams that apply a very different mindset to the problem or opportunity at hand.
What about empathy, for the customer and those doing the work to serve them? We must intentionally nurture the subtle, holistic awareness that we get as we go to gemba and observe the entire situation with all of our senses. With big data we can observe hidden patterns of consumer behavior, but talking to people — understanding how something positively or negatively impacts their experiences and their lives — affects us in an entirely different way.
And what about true, outside-the-box innovation? Some of the greatest leaps in human achievement have come when someone, in a moment of inspiration, looks at a situation in an entirely new context. Steve Jobs once said, “When you ask creative people how they did something, they feel a little guilty because they didn’t really do it, they just saw something.” How do we compute that? Shunryu Suzuki, founder of the San Francisco Zen Center, sums up the challenge: “In the beginner’s mind there are many possibilities, but in the expert’s there are few.” The same, perhaps, can be said for an expert system.
In this article, we do not present an argument against big data, cognitive computing, and whatever lies beyond. Clearly we must learn to improve the human-computer symbiosis if we are to harness this qualitative leap in understanding and improving the world around us. Rather, we argue for maintaining a healthy respect for the role of human capability and insight, recognizing that decision making must be a combination of technical and social aptitudes. Only by understanding the purpose of the organization, and the value it delivers to its customers both now and in the future, can we utilize this new technology properly. Both raw analysis and human creativity are necessary. They must be kept in balance, acting as catalysts for experimentation, continuous improvement, and innovation — the essence of Lean Thinking.
To make the right decisions, to ensure that we’re optimizing for the appropriate outcomes, we must look deep inside the situation, ask the right questions, infer the causes for the correlations, design the right experiments, solve the right problems, think outside the box, and develop empathy for our customer, while always reflecting on our purpose. We must ensure that, when engaging with big data, the guiding hand of human understanding, intuition, and empathy is always present in the management systems and the culture of the organization.
Acknowledgment
We deeply appreciate the efforts of a coauthor who must remain anonymous. His insights as a data scientist, Six Sigma Master Black Belt, PMP, and mechanical engineer, and his practice in the aerospace, nuclear, chemical, financial, and IT domains, have been very helpful.
1 This image was created by David Verble, former Toyota change agent and coach, and Lean Enterprise Institute faculty member, based on the original Purpose, Process, People work by Jim Womack and its later elaboration by Dan Jones.
2 These steps are consistent with two popular continuous improvement cycles: Deming/Shewhart Plan-Do-Check-Act (PDCA) and Six Sigma Define, Measure, Analyze, Improve and Control (DMAIC).
3 Basalla, Mark. Personal communication.