CUTTER BUSINESS TECHNOLOGY JOURNAL VOL. 30, NO. 7
This article examines the way the data collected over the IIoT ecosystem of interconnected humans and machines can be collected, managed, and exploited. The authors focus particularly on a concept they call "enterprise personal analytics" (EPA) and provide a digital transformation roadmap companies can use to adopt EPA, examining the emerging concept through different perspectives (company, worker, and modality) and related concerns (individual information systems architecture, knowledge and intellectual property, motivation and remuneration, information governance, and quality assurance).
Introduction
We’re in the age of auto-analytics, or the capturing and analysis of personal productivity data.
— Thomas H. Davenport
Organizations have long used analytics to improve performance. Indeed, research shows that top-performing organizations use business analytics five times more than lower performers do. In 2016 the business analytics industry was worth an estimated US $130 billion. It is predicted that industrial sectors such as discrete manufacturing, process manufacturing, telecommunications, and healthcare manufacturing will invest a combined total of $101.5 billion in business analytics by 2020.
One promising technological advancement in this regard will be the use of personal analytics. While traditional organizational intelligence metrics deliver a big picture of structures, processes, and roles, more detailed and personalized analytics enables employees to scrutinize their personal productivity in terms of their desired versus their actual way of working. Personal analytics empowers individuals to analyze and exploit their own data to achieve a range of objectives and benefits across their work (e.g., productivity, quality, performance) and personal lives (e.g., sleep, exercise, health). Personal data can relate to biometrics, personal finance, social media activities, health status, behaviors, emotional states, mobility, personal interest areas, and so on.
Organizational interest in personal analytics is also beginning to gain traction. In this article, we will focus on a concept that we call “enterprise personal analytics” (or EPA, for short), through which industrial organizations enable their workers to use their personal data to manage their digital working lives from descriptive, diagnostic, predictive, and prescriptive points of view.
Like many IT analysts, we believe that the emerging concept of enterprise personal analytics has the potential to become the new frontier of competitive differentiation. EPA may be of particular interest to industrial sectors such as manufacturing, utilities, energy, and aviation. For instance, EPA can enable skilled and unskilled industrial operators to analyze their own personal data to understand why they’re making the choices they’re making and then to combine their human expertise with the underlying objective data to create new operating procedures and processes. Wearable technology is increasingly being used in the manufacturing industry for employee safety, employee monitoring, video applications, field service, and plant monitoring. Organizations can also leverage the rich insights provided by nonverbal data — which can be captured by personal digital monitoring technologies for time management (e.g., Microsoft MyAnalytics), facial coding (e.g., Affectiva, Microsoft Emotion), brain imaging (e.g., NeuroSky, Emotiv), pupillometry (e.g., Tobii, Eye Square), and physiological monitoring (e.g. Empatica, Fitbit) — to improve efficiency and attention management, increase well-being, and reduce mistakes. Industrial organizations can use all of this EPA data and more to provide actionable insights that directly support their most important business decisions (automating a process versus losing employees’ tacit knowledge, rewarding star players/teams, enhancing the physical and mental well-being of employees, etc.).
The EPA Digital Transformation Roadmap
The use of personal analytics in an enterprise setting is different from its use in other environments (e.g., private use). This has implications for which aspects of personal analytics should be considered in an enterprise context. We have identified five specific concerns pertaining to the use of personal analytics in an enterprise setting: individual information systems (IIS) architecture, knowledge and intellectual property (IP), motivation and remuneration, information governance, and quality assurance. As EPA involves a number of stakeholders, it is useful to study the concept from different perspectives. Our analysis has revealed three relevant perspectives: company, worker, and modality (i.e., the mode through which companies enable their workers to use personal analytics). Consequently, we have used a two-dimensional grid (concerns vs. perspectives) to define a roadmap that organizations can use to guide their EPA digital transformation efforts (see Table 1).
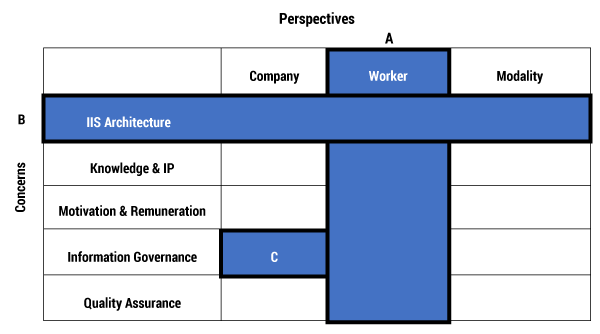
Table 1 — The EPA digital transformation roadmap: (A) a multi-concern, single-perspective view;
(B) a single-concern, multi-perspective view, (C) a single-concern, single-perspective view.
Concerns
IIS Architecture
IT consumerization, or the adoption of consumer devices and applications in the workforce, is pervasive. Employees bring computer tablets and smartphones into the workplace and harness social media applications and special-purpose apps for their work lives.
According to Georgia State University Computer Science Professor Richard Baskerville, a typical IIS architecture consists of two specific work systems: the individual’s work system as an employee, and the individual’s work system as a person. These systems are facilitated by individually and enterprise-provided cloud computing technologies, which produce and consume services.
Figure 1 depicts an employee’s IIS architecture. Given the opaque nature of IIS, our knowledge of how the employee has designed and planned the architecture and how he controls it is limited. Why has he made the choices and investments reflected in Figure 1? How could the organization optimize his IIS architecture? Baskerville opines that organizations can no longer ignore IIS architectures for the following reasons. First, IIS “represent the most recent frontier for the design of computer-based [information systems].” Second, IIS are “complicated and unique systems that cross the boundaries” between personal life (e.g., social aspects) and work life (e.g., organizational aspects). Third, IIS do not merely store data; individuals are “actively collecting data and processing it into information for various purposes and feeding it outward.”
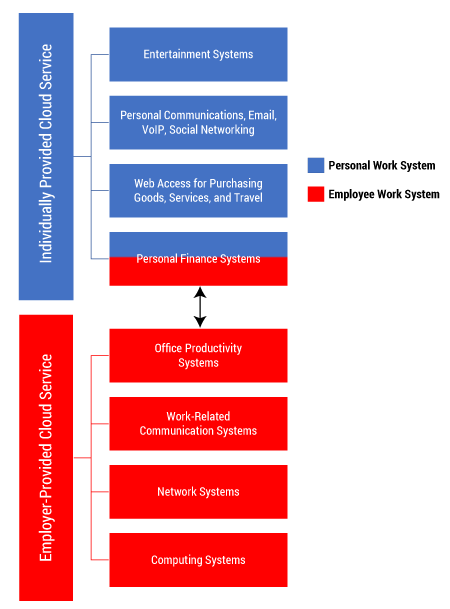
Figure 1 — An employee’s IIS architecture. (Adapted from Baskerville.)
Knowledge and IP
With the aid of digital technology and the tracking and monitoring of the self, optimization becomes not only possible but also desirable. In an EPA context, where the individual worker’s personal analytics data is viewed as an information asset, the manner in which knowledge and IP are managed becomes of paramount importance from a legal perspective. For instance, when an employee leaves a company, who owns the individual worker’s EPA data — the company or the worker? If the latter, can the worker use her own personal analytics data to highlight her expertise and skills to prospective employers in a sort of digital résumé? Would this transfer of EPA data make companies more vulnerable to competitors employing collective intelligence techniques (e.g., using competitor data sources to predict their strategies)?
It may be that there are irreconcilable conflicts between a company’s and an employee’s interests with regard to EPA. Take, for instance, cases where organizations make employees sign noncompete agreements, which can hinder the employees’ ability to take their personal data to another job in their current industry. For EPA initiatives to succeed, organizations must be sensitive to employees’ concerns, which can be alleviated through education and transparency. One possible solution would be for organizations to create BYOD policies that encourage workers (e.g., by providing financial support) to purchase and bring their own monitoring devices to work. In such an arrangement, the workers would have full control of their own data. For example, Philips has developed the Rationalizer EmoBowl and EmoBracelets for stock traders. These products are designed to feed data solely to the individual workers (e.g., the primary consumers), with no executive involvement, so that they can alter negative working patterns and enhance productivity. Further EPA business cases are required to determine how companies can effectively implement a requisite level of knowledge production versus knowledge protection in an EPA context.
Motivation and Remuneration
EY Global Chief Analytics Officer Christopher Mazzei observes that “how an organization measures and rewards employee performance matters,” underscoring “the importance of aligning incentives with desired behaviors” in the context of personal analytics use.
Because meaningful individual analysis can only be achieved after an adequate volume of data has been collected, the choice of EPA data analysis medium is an important consideration. In a personal analytics context, “employees may look into their data with different goals, backgrounds, and expectations (e.g., internal context). However, the vast majority of people are not visualization or data analytics experts, so analytical tools will need to be accessible,” note University of Victoria researcher Dandan Huang and her coauthors. It is recommended that organizations opt for technologies that come with minimal learning overhead. Ultimately, organizations must develop EPA strategies that inspire long-term use amongst workers.
That said, there is a gray area with respect to how continuous self-monitoring of one’s personal analytics can impact workers. For instance, UK researchers concluded “it is conceivable that people may become over-reliant on automated systems that provide a false sense of security or ... could also suffer from negative consequences of excessive self-monitoring by finding it uncomfortable, intrusive, and unpleasant.” Workers may also be reluctant to share their personal data openly with peers, particularly when that data is used to compare their individual performance with others on their team. The lessons learned from the large-scale abandonment of personal health–tracking technologies, which is currently occurring amongst users of smart watches and fitness trackers, can provide valuable insights. One study found that “features that signal the device’s ability to collect activity data are essential for adoption, whereas device portability and resilience are key for sustained use.”
Information Governance
Information governance is the set of multidisciplinary policies and controls aimed at managing information at an organizational level, supporting legal, regulatory, and risk compliance requirements. Effective information governance policies secure confidential data and enable unneeded data to be disposed of in a systematic and legally compliant manner. The potential nexus of parties (e.g., partners, workers, customers, data pools, cloud and network providers) encompassed in an EPA initiative necessitates robust information governance mechanisms. Specifically, the European Union’s General Data Protection Regulation (GDPR), which comes into force in 2018, applies to all companies worldwide that process the personal data of EU citizens. GDPR will introduce:
-
A broader definition and scope in relation to personal data
-
Stringent worker consent procedures
-
Mandatory privacy impact assessments
-
The appointment of a data protection officer
-
Common data breach notifications
-
Stringent data-handling principles (e.g., the right to be forgotten)
-
Privacy by design requirements
-
A tiered financial penalty structure
Industrial companies considering implementing EPA initiatives will have to operationalize information governance strategies that are fully in line with GDPR requirements.
There may also be a need to develop regulatory frameworks that support the validation of EPA initiatives. Such frameworks could potentially persuade enterprises to collaborate within a community of organizations with a vested interest in providing open access to their collective personal analytics methodologies and data collection and analysis protocols. Similar approaches have already been effectively adopted in the healthcare industry, where manufacturers of wearable technology devices have established interconnected open source platforms where information can be exchanged in order to ensure the reliability of the devices while also alleviating security and privacy concerns. Another example is the Connected and Open Research Ethics (CORE) initiative, which aims to bridge the gap between manufacturers, researchers using wearable technologies, and ethics boards tasked with protecting the research participants with respect to the processing of personal data.
Quality Assurance
Personal data is characterized by unpredictability and high volume, variety, and velocity, and the value of this data to an enterprise will be commensurate with the data quality and the power of the analytics done on it. Many enterprises have reached a point where their ability to generate data exceeds their ability to consume it. Furthermore, they have merely developed the capability for analytics production in the absence of any analytical tools that can provide significant insights.
Data visualization tools (Tableau, Raw, DataHero, etc.) have been lauded as a means of addressing this disconnect. With regard to EPA, we encourage industrial organizations to develop new methods, tools, and principles for ensuring data quality, reliability, and certainty throughout the data transformation and analysis process. New tools are also required to support personal data analysis in order to make insights easy to comprehend and actionable at every point within an organization and at every skill level, from experts to analytical amateurs.
Organizations may find that there are many data sources that can be better and more easily analyzed through their enterprise BI analytics tools, as opposed to the less robust analytical capabilities and limited data sets on workers’ individual mobile and wearable devices. If that’s the case, workers might be more willing to use EPA in their working lives in order to derive more meaningful results.
Perspectives
Company
Companies can have different motivations for using EPA. The use of personal analytics has much to teach organizations about alignment, performance improvement (individual and team), and business ecosystems. For example, a company may want to increase innovation in its current business processes. The use of personal analytics can enable employees to identify and suggest improvements that can result in cost savings, better customer service, and decreased employee frustration. These new improved processes and follow-on developments can become institutionalized across an organization. Furthermore, companies can derive value from employees who use their personal analytic devices (e.g., smart watches) to productively sync to organizational resources while working remotely.
In most businesses, “analytics have typically focused on operational or marketing issues and not on the human dimension of performance…. Even when companies do employ human resource analytics, their approaches are not sophisticated and have been applied only to individuals,” writes Babson University Professor Thomas Davenport. Yet there is also scope for organizations to use EPA in a team context so that they can evaluate performance across different team compositions. Davenport discusses how companies, employing a technique called “plus/minus” analysis, can use personal analytics to assess not just individual performance, but the way specific teams perform with or without a particular worker.
Before launching an EPA digital transformation initiative, however, there are a number of considerations a company must take into account. These include identifying the maturity and sophistication of the organization’s analytical capabilities, determining the turnaround time for implementing such a strategy (e.g., quick wins vs. longer-term goals), implementing procedures for gaining strong organizational commitment toward the new EPA strategy, and, most significantly, considering the impact on individual worker morale and team dynamics.
Worker
The use of personal analytics can also benefit workers by increasing their understanding of how their work impacts the company’s strategy and goals (e.g., business insight), facilitating meaningful working environments, enhancing career opportunities, and improving job satisfaction. These factors are critical for retaining and engaging all types of workers. For instance, analytics expert Jeanne Harris and her coauthors found that the use of modern digital technologies in the face of “changing characteristics of the generation of employees now entering the workforce, particularly their high levels of comfort with, and expectations about, social networking and consumer technologies ... was seen as a valuable tool in attracting and retaining these new hires.”
Companies require a better understanding of how to best operationalize EPA efforts within the organization at an individual worker level and assistance with the core analytics processes that would be required to support this. For example, Davenport describes a scenario in which a company enables its sales employees to use the extensive data from its customer relationship management applications in order to assess and improve their performance. If, for instance, the most successful sales professionals tend to spend less than 10% of their time on lead generations, then average and low performers could adjust their daily work routines to do likewise.
Modality
Modality refers to the mode through which personal analytics is experienced by the worker or is deployed by the company. This is becoming an increasingly important perspective, as intelligent digital ecosystems are being supported by a fluidly and dynamically interconnected mix of:
-
Individuals
-
Information
-
Services
-
Devices, such as:
-
Traditional computing and communications (platform, desktop, mobile, tablet)
-
Wearables (health monitors, augmented and virtual reality displays)
-
The Internet of Things (consumer appliances, transportation, and environmental sensors)
-
Data storage (hard drives, cloud, USB)
-
As our digital environment evolves, a number of important questions emerge in the context of EPA modality. For example, how does the user experience fundamentally change, and what digital technologies, security architectures, and platforms are required to support this change? Most significantly, the ability for workers to use multiple modalities effectively (which will entail multi-tasking and context switching) is a salient requirement for the successful development of a company’s personal analytics strategy. Therefore, when contemplating the best modalities for their EPA initiatives, organizations should consider the frequency of interruptions and how quickly users can recover from them.
Using the Digital Transformation Roadmap
Having examined the five core EPA concerns and the three perspectives, let’s take a look back at the EPA digital transformation roadmap depicted earlier in Table 1. A novel feature of this “grid”-type roadmap pertains to the various pilot study designs that can be operationalized. The first design is a multi-concern, single-perspective view denoted by the letter A. The second design is a single-concern, multi-perspective view denoted by the letter B. The final design represents a single-concern, single-perspective view denoted by the letter C. Given the rather infant status of the EPA phenomenon, we encourage future organizational pilot studies to populate each of the cells within this grid framework. To assist the process, we have devised a number of questions that we believe merit further consideration (see Table 2).
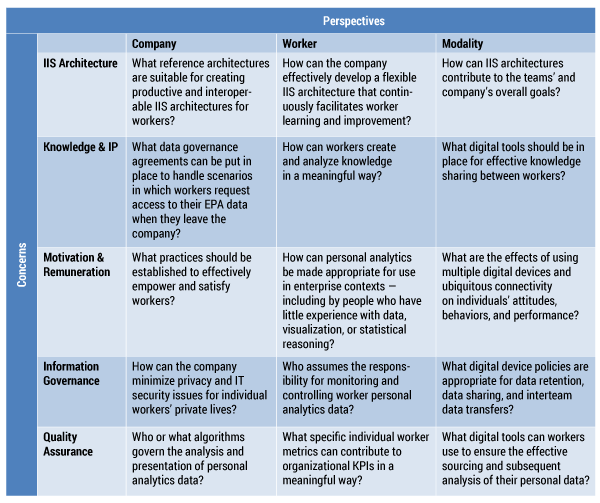
Table 2 — EPA digital transformation roadmap example questions.
Furthermore, there is a significant need for EPA business use cases and EPA adoption frameworks. In our analysis, history suggests there are two dimensions that impact how a disruptive technological trend and its business use case evolve. The first is complexity, which is represented by the level of coordination required by all parties in an organizational ecosystem in order to produce value with the technology. The second dimension is novelty, which describes the level of effort a user requires to understand the problems that the new technological trend can solve. The more novel a concept is, the greater the learning curve.
We encourage industrial organizations to develop adoption frameworks that map possible EPA implementations against these two dimensions. Complexity and novelty can vary from low to high in terms of the stage of technology development. For instance, industrial organizations new to the EPA concept may want to introduce an EPA pilot program that is low in novelty and low in complexity (e.g., use of wearable fitness trackers to reduce the organization’s insurance policy premiums).
In order to advance the emergence of EPA business use cases, we have also devised a visual mapping artifact that we have coined the “EPA digital transformation metro map” (see Figure 2). It depicts possible routes that companies must navigate through the five concerns across the three perspectives raised in this article:
-
Pink = IIS architecture
-
Blue = Knowledge and IP
-
Yellow = Quality assurance
-
Red = Information governance
-
Green = Motivation and remuneration
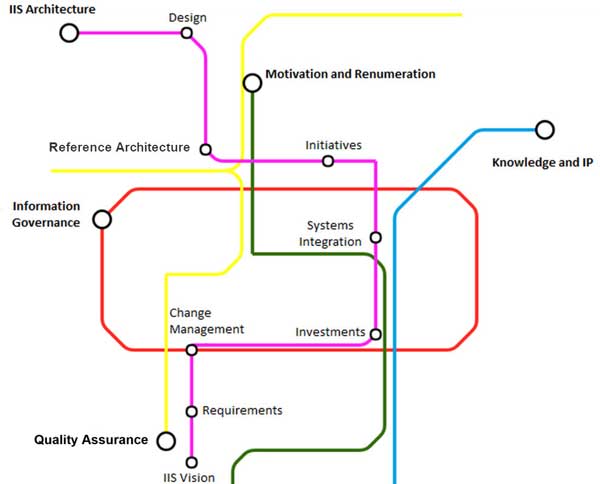
Figure 2 — EPA digital transformation metro map.
For illustration purposes, we have completed the journey for the IIS architecture concern (i.e., the pink route). This specific journey comprises possible “route stops” that organizations must consider in terms of:
-
Design (e.g., security, applications, and process architectures)
-
Reference architecture (e.g., business continuity management, analytics sources)
-
Initiatives (e.g., flexible architectures to ensure continuous learning)
-
Systems integration (e.g., legacy systems)
-
Investments (e.g., wearable technology vs. desktop monitoring)
-
Change management (e.g., new employee onboarding)
-
Requirements (e.g., individual vs. group)
-
IIS vision (e.g., alignment with the main EPA strategy)
We must stress that we have only used these specific route stops for illustration purposes, and we encourage industrial organizations to carry out pilot EPA projects to identify the specific stops that are the best fit for them with respect to all the concerns outlined above.
Conclusion
Global businesses are increasingly embracing the use of personal digital technology in the workplace. Wearable technologies, for example, are providing capabilities that are completely new to mainstream business practices (e.g., Tobii’s EyeX, SMI eye-tracking glasses). While the application of EPA in an industrial setting remains a relatively niche practice, we believe its impact will fundamentally change the industrial landscape. Ultimately, industrial sector organizations will be at the forefront of the EPA drive with, according to Davenport, “the bulk of data from emanating sensors within an ‘the industrial Internet,’ comprising a very large number of networked devices in plants, transportation networks, energy grids, and so forth.” We believe that if organizations behave appropriately and build a culture of trust, companies and workers (and customers) will become acclimatized to capturing and analyzing their personal data within an enterprise setting. Organizations need to proactively prepare themselves for this change, as EPA is coming soon to a company near you!