CUTTER BUSINESS TECHNOLOGY JOURNAL VOL. 31, NO. 9
Martijn ten Napel, a practicing architect in the field of business intelligence (BI) since 1998, explores the challenge that has confronted him throughout his career: why do so many BI projects fail? His conclusion is that the struggle to achieve coherence between people, process, information, and technology has caused the complexity of the data landscape to grow out of control. His answer to the problem is the connected architecture — a framework and thought process for the organization of DW and BI projects. He believes it applies equally to digital business.
Digital transformation is not so much about the use of technology. Instead, it concerns creating fluidity in what used to be demarcated boundaries between stakeholders in a business process. Digital information flows enable fluidity. Direct communication with consumers along with a quick adaption to their shifts in taste — or fine-tuning to individual preferences — are changing the way businesses operate.
Technology has enabled us to build systems that support business processes across the boundaries of physical and legal entities. Technology has resulted in a restructuring of market conditions. Consequently, the competitive space has been altered and businesses are adjusting to this change.
But adjusting isn’t easy, and information is not a fixed stream. The information itself and where it originates are dynamic. The process of pulling it together, deriving insights from it, and acting upon those insights is quite a challenge. Attempting to achieve the desired business agility — an attempt that will never reach a final state — is hard work.
As a result, businesses need to significantly adapt the way they govern their information landscape. The old demand-supply model of IT governance isn’t adequate; we need a governance model that accommodates extreme fluidity.
Riding the Data Beast
Agility is one of the key objectives in a governance model that accommodates fluidity. Agility is a result of the culture of an organization, not a state that can be achieved by implementing the rituals of an Agile working method. The foundation for this statement comes from the observations I have made and the experiences I have gathered over 20 years across a diverse set of businesses. It is difficult to reach a state of agility that is sufficient. There are many reasons why this is difficult, but I observe one common theme: universally, people struggle with the data beast. Putting information to work in a way that is productive is like riding a rodeo bull, whether you call it business intelligence (BI), business analytics, data warehousing (DW), big data, information management, data governance, or digital transformation.
Putting information to work for your business is a collaborative effort; an effort that is a continuous process, not a project with a finite result. Every time you feel you have obtained a definitive result, the data beast starts to buck again.
So what is this beast and why does it buck?
What Is the Data Beast?
The collaborative effort is a consensus model. You and other stakeholders need to discuss and arrive at a shared conclusion of what information means to you and how to adjust a business process. The adjustment can change the way a business process runs or lead to process redesign in digital businesses.
Information gathering and recording are plagued by fragmentation, context switching, and volatility. These problems seem to be inherent to working with data and constitute the data beast. The contradiction between, on the one hand, the search for consensus and, on the other, the fragmentation, context switching, and volatility of information that dilute this effort (see Figure 1) is a never-ending rodeo ride.
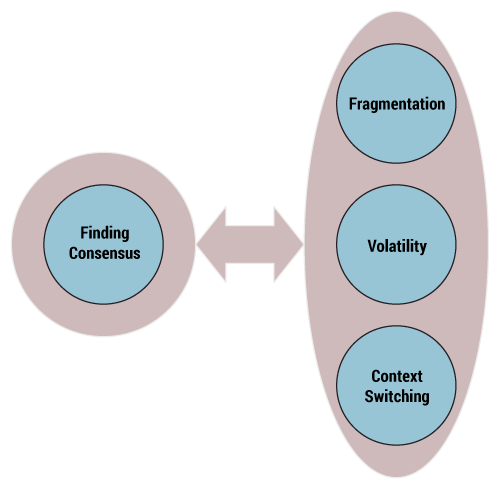
The reasons behind fragmentation, context switching, and volatility are misunderstood. This triple plague is often seen as either an imperfection that requires fixing or a roadblock to digitization. As a result, the way we deal with it is often counterproductive.
Before diving into this problem and suggesting how you can rethink the challenge of digitization, let me first explain what I mean by fragmentation, volatility of information, and context switching.
The Nature of the Beast
Fragmentation
To get the whole picture of the business, information must be assembled from different software systems or data stores. This is fragmentation. In DW or enterprise application integration, this phenomenon leads to the need for integration.
With digitization, information is becoming increasingly fragmented. Connectivity, the driver of digitization, enables us to compose business processes across supply chains and across cloud services. Information is not integrated but rather connected across the steps in the business process or the particular services that deliver one or more functions of the business process. For instance, Salesforce.com can be used as a service within different business processes. It enables the recording and retrieving of customer interactions across processes or across the different communications channels and social media outlets that engage customers, but its data set is isolated from the data sets in other parts of the business process. Consequently, keeping the definition of information in sync is a challenge.
Disparity of data is not the only consequence of using a service. Loss of ownership has an even more profound impact. For instance, think about the message service WhatsApp. For many businesses, it plays an important role in customer interaction, but its functionality is determined by Facebook, not by the business using it. If a business composes a business process from different services it does not control, fragmentation makes it harder to keep information consistent throughout the business process as opposed to when a process is composed from systems the business controls.
Volatility
The volatility in the information landscape is increasing because businesses are interacting and assembling their processes in different ways; for example, by enabling and switching to cloud services. Cloud services offer new functionality at an ever-higher velocity to remain competitive. Maintaining information consistency is hard work when the landscape in which data is recorded changes all the time.
Since services are for the most part no longer owned by the businesses that use them, businesses must keep up with the velocity set by the service provider. Many companies struggle with this externally driven change in IT operations. Businesses cannot plan for this change; they must simply keep up. People using and retrieving information from these services are no longer in the driver’s seat but are merely passengers along for the ride.
Fragmentation and volatility are not exclusive to DW practice, but data warehouses are often the systems where the volatility across all systems converges and where fragmentation has a significant impact on being able to keep information consistent over time. The BI community has learned how to deal with this, and those methods can be applied to the digitization of business processes.
Context Switching
Context switching occurs when information is used outside the context in which it was recorded. It is what happens when you ask the question, “What does this piece of information mean?” A simple example can be found in a financial transaction that leads to an entry in a financial ledger designed to record the transaction. When you aggregate financial information across ledger entries and ask the question, “Are we on track with our sales goals?” you interpret the ledger entry in another context. Depending on the answer, you might follow different courses of action.
Context switching is the least understood and hardest part of working with information. It is where consensus on how to define a shared context for use of information must be found. What makes DW difficult is that you must embed this consensus on meaning, in the context of a particular use, in your data models.
Most DW practitioners have rejected the adage “one version of the truth” and have replaced it with “one version of the facts; multiple views on the facts.” It is an acknowledgment that the same data point, recorded for a specific purpose, can have different meanings in different business contexts.
Context switching is a phenomenon that used to be confined to data modeling in the DW and BI functions. Connectivity, together with the low cost and massive growth of compute power, has made it possible to integrate the decision-making process directly into business processes. As such, the challenge of context switching is dispersed across the whole business and is no longer an exclusive BI or analytics phenomenon. Dealing with multiple contexts of use is another lesson that can be carried over from DW to the digitization of business processes.
Context switching is where the data beast is really trying to throw you off its back. With the digitization of business processes and the fluidity in the boundaries of a business, the number of contexts in which information is interpreted has grown enormously. When finding consensus, or automating decisions within a digitally enhanced business process, you must be very careful to understand the context of the participating actors.
And here is the tricky part: consensus on “What does this piece of information mean?” will often lead to a next action. That next action might lead to a change in the business process or in the context of the next question (a new or adjusted interpretation). BI professionals know that people can only tell you what information they need once they have seen it. Insight gathering and achieving consensus make for an interactive and progressive change process that often leads to more volatility and, if you are not careful, increased fragmentation.
Taming the Data Beast
How to deal with these phenomena — fragmentation, volatility, and context switching — is where opinions start to diverge. The most common approach is to strive for unification and to create standards and standard services.
Most people agree that volatility is a given. Many architects will reason that defining standard components or standard building blocks is a precondition for achieving agility in composing or changing business processes. The line of thought is that standardized components can be swapped in and out when necessary, with a short time to market, low cost, and limited impact on other standardized components. This is the engineering point of view, comparable to a builder using standardized components in different house designs that can be chosen from a catalog.
I’ve been advocating this approach for a long time but have never arrived at a satisfactory state where this standardization led to a smoothly running and agile information stream. On the contrary, the more volatility popped up and the more “standard” solutions were introduced, the harder it became to reach consensus and deliver fitting information solutions. What I’ve noticed is that using standardized building blocks in IT architecture always increases information demand. People miss information that was disposable in the customized solution they use, and they try to find other ways to get to that information. Part of the demand is the human aversion to change and projects not taking care of educating the users, but part of it is also IT people making decisions on priorities without fully understanding the business context of users. With increased volatility, the information demand rises beyond what a company can cope with and people start to improvise and create local solutions. As a result, fragmentation increases exponentially.
In my DW and architecture practices, I’ve tried different ways to approach a solution, taking what I’ve learned from other projects. Moreover, I’ve been a proponent of using Agile working methods to deal with the learning curve resulting from context switching. Such methods enable us to take steps forward in maturing the process of working with information.
Maturity is the result of two processes being managed in an appropriate fashion. One is the collaboration process between information users and information provisioners, to better understand the business context of users, but also for users of information to understand what data can and cannot do for them. The other process is the prioritization of new information requests based on business value. This requires users to collaborate, to see beyond their own business context, and to be able to decide what is of more value to the entire organization. Agile working methods work, and when implemented the right way, I’ve seen consistent improvement. But it is not enough.
Time and time again, fragmentation and volatility complicate this process. Reaching higher maturity levels in creating consensus out of information and in acting on this consensus is challenging. Often, businesses slide back after a while until a new manager tries again and initiates a new project or a new change. This has been puzzling me for a long time. We know the problem, and we brought along our data architectures and our ways of working as a BI community to offer solutions. Why are we failing to make our practices stick?
Changing Our Approach to the Data Beast
What We Can Learn from DW Practice
I believe we have been denying the true nature of the data beast. I am convinced that we have misunderstood the causes of fragmentation and volatility. We have regarded them as imperfections that we have to battle, instead of as artifacts of our own behavior.
Put DW architects together in a room and the discussion turns quickly to the best way to deal with both fragmentation, which leads to integration challenges, and volatility in the information landscape over time. Now, many architectures are available, but there is a tradeoff around when to integrate and what it means for the changeability of information. The common complaint I’ve heard in 30 years of DW has been “Why does creating, extending, or changing the data warehouse take so long?”
The answer is that dealing with fragmentation, volatility, and context switching is hard work and it needs close collaboration with the users of the information. Fragmentation and volatility drive the need for a consensus-achieving process and are, at the same time, the results of the consensus-achieving process. Using information and achieving consensus on its significance through discussion leads to a next action. The results of that action could well be a change to the business process and the supporting IT systems. It could even lead to a redesign, where parts of the process are reimplemented in services or new parts are added to the business process, sourced by a new supporting IT system. In turn, changed or additional information needs to be collected from these new or changed systems or services. Context switching complicates our understanding of, and our reaction to, dealing with fragmentation and volatility. Test, experience, observe, debate, and adjust are how we humans progress in business. With the digitization of businesses, this challenge intensifies as volatility increases.
In digital businesses, fragmentation is a design decision to deal with the fluidity of the business processes and business boundaries. Understanding the consequences in terms of the energy it takes to keep information consistent across a fragmented data landscape is among the key DW insights to be carried over.
Dealing with the Data Beast
Once you can accept that the beastly nature of working with information is created by your own actions and is an inherent part of the collaboration process that makes information work for you, you can finally start to formulate solutions.
One lesson to take from DW practice is to never assume a future state. The information landscape will always be fluid. Volatility is a given. At the same time, we must deal with decisions made in the past: historical patterns in data cannot be undone. As a discipline, we have developed coping mechanisms.
Architects have formulated concepts like loosely coupled services to create connectivity between the components of a business process and its master data; that is, to integrate the components into a coherent process. The scale at which loosely coupled services are deployed has changed, and the ownership model certainly has changed with digital transformation.
Fragmentation isn’t bad; it can be used to create the conditions to achieve agility in the data landscape if it is used as a design principle. It allows you to string together software services or components. Volatility and fragmentation across different software components are expressed in fragmentation of master data. Acquisitions and mergers, partial replacement of ERP systems for cloud services, and changes to business processes result in fragmentation of master data.
When we talk about context switching and a need to reach consensus, master data is the integration point. Fragmentation makes integration harder. On the other hand, having multiple expressions of the same master data item is not an unfortunate side effect of context switching, but the result of having to deal with multiple contexts. Multiple expressions of the same master data item might even be inevitable with the increasing fuzziness of business boundaries.
My experience at Free Frogs, a cooperative BI company, has shown that applying the principles of “loosely coupled” to master data and containing fragmentation within a framework that governs the collaboration process will lead to the design patterns of solutions that fit this collaboration process. This is designed fragmentation, or “connected architecture,” as dubbed by Free Frogs (see Figure 2). This framework is a thought process more than a recipe.
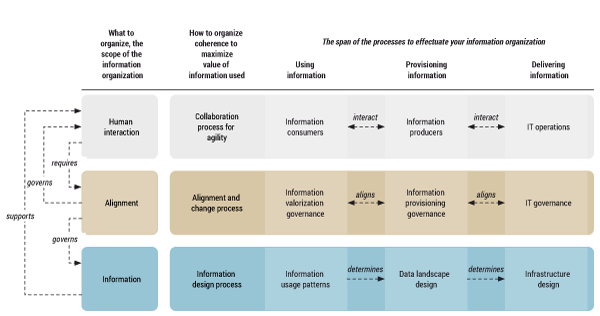
Connected Architecture in Designing the Arena for the Data Beast
Human interaction is key. This is the first line in Figure 2. Working with information requires a collaborative process involving all disciplines in order to facilitate reaching consensus on the meaning of information in its various contexts and to determine the actions to be taken. In other words, context switching is a learning process that needs close collaboration among three groups of people with different skills and different responsibilities: those who use information and create value out of it, those who have the analytical skills to provision and interpret the information for users, and those who have the skills to prepare the information and who can automate the data streams involved. Close collaboration needs reinforcement into solutions to align the business process to the consensus.
The process of alignment, the second line in Figure 2, requires an architecture process that reevaluates the design continuously and adapts to the volatility, thus enabling agility. Architecture cannot create agility, but architecture can create the conditions for agility to thrive.
You need to focus on what brings value. Most information has limited value, but you need the process of finding consensus to determine which information is valuable. Of course, what is valuable changes over time, and volatility also changes the value of information. You need a guided process to focus the effort of collaboration on what has value. It won’t happen by itself; you must organize the collaboration process. The architecture process must find a delicate balance between agility and alignment.
This implies that the architecture process shapes the information design process — the third line in Figure 2 — which uses fragmentation as a design principle to safeguard the required agility. Where this agility is needed emerges from the collaboration process. We know these needs are not stable by the nature of the collaboration process.
This task of finding balance is not simple. An architect cannot get it right all the time. Like all people involved in the process, you learn from the collaboration, from inspecting the results, and you build and change both the landscape and the conditions for the human interaction process. Your design process must be incremental, and your data landscape design should be able to facilitate the evolution of insight. This evolution originates in the outcomes of context switching.
Once you accept that fragmentation, volatility, and context switching aren’t disruptors to digitizing your business processes but instead are ingredients to create solutions that support them, it becomes much easier to wrap your head around what is necessary.
This way of thinking originates in dealing with the challenges caused by fluidity in the information that flows to and from a data warehouse. These challenges are not unique to DW and the framework can be applied to other parts of the digital business as well.
The governance model needed to accommodate fluidity is challenged by the nature of working with information. Once you have more knowledge of what this nature is, you can adapt your information governance model to work with that nature instead of trying to battle it. It is impossible to deliver guidelines on how to do this in the scope of a single article, but you can take a second look at your digital transformation efforts with the following three takeaways in mind:
-
Take a critical look at who is represented in your digital transformation teams. Is the mix of users, business process domain experts, information professionals, and IT professionals balanced? Do they collaborate on equal terms?
-
Hire a few architects who understand the nature of working with information and are equipped to guide the teams. They should blueprint your digital transition, paying careful attention to the balance of the level of fragmentation in the data landscape, but leaving the details up to the teams.
-
Incorporate the professionals of your DW or BI departments into your digital transformation teams. This helps to define integration patterns and deal with different expressions of the same master data item across different systems.