CUTTER BUSINESS TECHNOLOGY JOURNAL VOL. 32, NO. 8
As AI becomes more visible as a corporate strategic tool, organizations will have to incorporate issues surrounding AI as part of corporate strategy. Pavankumar Mulgund and Sam Marrazzo help us by providing a framework for developing an AI strategy. The authors discuss the “minimum viable model” approach to the development of the underlying AI/ML models, along with the platform on which these models run and the inevitable tradeoffs. They conclude their piece by examining some best practices for the successful implementation of AI initiatives.
It has been widely reported that the lack of an artificial intelligence (AI) strategy presents the most significant hurdle to the implementation of AI initiatives in any organization. While most companies appreciate the need for a company-wide AI strategy, they often struggle to articulate a well-thought-out future course of action that takes various strategic considerations into account. Development of such an actionable plan is difficult as it depends on a variety of contextual factors that are unique to every organization, such as the organization’s digital maturity, executive buy-in, the skill and experience of team members, and resistance to change.
Yet many companies, digital and traditional, are adopting AI technologies, driven mostly by the fear of missing out. Consequently, many investments in AI have been made as one-off implementations led by a visionary team leader, as opposed to a systematic and organization-wide strategy, although this trend seems to be changing.1 Another challenge to the articulation of AI strategy is the emergence of a plethora of new terminology and buzzwords with no consensus as to their meanings.2 Moreover, there is a considerable dearth of talent in the AI space. Plus, there is a minimal supply of leaders who can translate organizational business priorities into a roadmap for AI initiatives and facilitate a conducive environment suitable for the successful execution of those initiatives.
In this article, we address some of these salient issues by providing a framework for developing an AI strategy. At the outset, we define a handful of the essential elements of AI strategy. Next, we explain our framework, which not only identifies and discusses critical success factors of an AI strategy but also presents a traceability map between business objectives and corresponding technology-related decisions. Following that, we present the gradual process of developing AI capabilities within an organization and highlight some of the inevitable tradeoffs in the real-world context. Finally, we draw heavily from the cutting-edge ideas and perspectives of thought leaders in the field to provide readers with a shortlist of best practices.3
AI: What Is It? Why Now? How Big Is the Opportunity?
AI has been around for more than half a century. A team of illustrious scientists and engineers first coined the term in 1956, and their intended meaning was “to make machines use language, form abstractions and concepts, solve kinds of problems now reserved for humans, and improve themselves.”4 Contemporary AI has undergone a considerable transformation and includes machine learning (statistical machine learning and deep learning), symbolic learning (computer speech recognition and vision), and robotics. These underlying methods, along with the emergence of sophisticated hardware components, have not only helped achieve human-like decision-making ability but also the capability to carry out a variety of complex operations. Naveen G. Rao, director of Intel’s AI Products Group, notes that “we are now entering the age of AI, a time when machines are using algorithms that give them superhuman abilities.”5
While AI technologies have captured the attention of computer scientists and academics for over half a century, there is now an unprecedented commercial interest in AI technologies. Several factors have influenced this exceptional interest. First, technological advancements in data storage and cloud computing, along with technologies like Hadoop for data mining, have increased the availability of data. In addition, with the emergence of graphics processing units (GPUs), the hardware is now available to process large volumes of data on a real-time basis from sources such as streaming videos, machine learning (ML) data sets, and various Internet-based wireless sensors. It has therefore become viable for organizations to pursue commercial products leveraging AI technologies. Market researchers are estimating today’s AI opportunity to reach nearly $78 billion by 2022.6 With such immense market potential, many industry leaders tout AI as enabling the next wave of innovation, similar to the role of the Internet in the early 2000s.
Many companies are keen on being at the forefront and capitalizing on the opportunity. Therefore, organizations, small and large, are making massive investments in the AI space. However, as with all prior technology bubbles, only a small subset of companies will make a substantial profit from these investments. For the rest, it will be an expensive strategic error at best or an existential threat in the worst case. In what follows, we discuss the first steps in terms of strategy and implementation to make the most of the AI opportunity.
What Is AI Strategy? What Are Its Fundamental Components?
The term “strategy” is defined as a high-level plan that an organization follows to achieve sustained competitive advantage. Therefore, AI strategy, at its core, must address vital questions, such as the following: How can AI deliver better value to customers? How can it help companies increase revenues, enhance efficiency, and reduce human errors? How can AI capabilities be integrated into the existing organizational processes to develop a distinct competitive advantage?
To address these questions, AI strategy must closely align with a company’s business objectives, ensuring synergy between the corporate strategy and the AI strategy. As illustrated in Figure 1, the strategy development framework begins with executive leadership identifying various strategic business outcomes. Increased revenue, reduced costs, and improved product performance are some examples of business value measures. Next, both technical experts and business leadership collectively prioritize digital and traditional process flows that have the highest potential to positively impact business outcomes. Thus, business leaders should identify workflows and use cases that bring the highest business value to the company with the least investment. The strategy team should make a quick evaluation of these use cases in terms of ROI to shortlist early candidates for implementation. Subsequently, both technical and business leaders should explore how they can embed AI within current digital and traditional workflows. While AI can augment some processes easily, others might require more disruptive transformation.
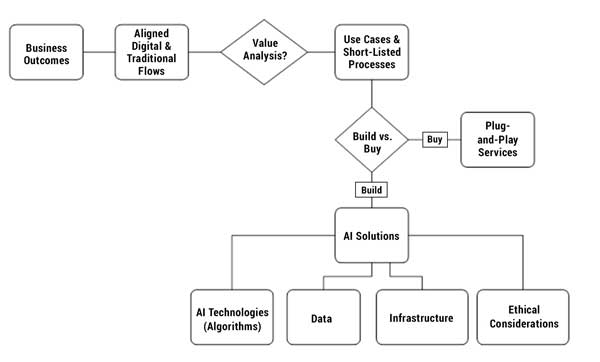
Another vital consideration in AI strategy is the issue of build versus buy. The leadership and technology teams should carefully review whether they should build a comprehensive on-premise AI platform or buy plug-and-play AI services from other platforms. It is prudent to leverage the AI platforms of other companies, especially in the early phase of AI adoption, as it reduces the need for enormous up-front investment and generates quick wins that can be instrumental in acquiring executive support. Typically, companies develop a minimum viable architecture using third-party vendors such as Microsoft, Google, and IBM. As the organization’s digital maturity increases, however, it should revisit the need for an AI platform. Moreover, while the organization should develop AI capabilities incrementally, it should produce an enterprise-wide vision for AI as early as possible.
In addition to alignment with business objectives, an AI strategy must consider four critical components: data, infrastructure, algorithms, and ethical considerations.
Data
Data is essential for the success of AI initiatives and is more powerful than the underlying algorithms that use the data. Companies typically perform data collection, cleansing, and preprocessing activities to ensure high data quality. However, these activities are challenging, as the data is usually fragmented across different silos, is inconsistent in format, may contain noise, and may be missing values. The digital industry has witnessed several examples where the poor quality of data has adversely affected some very sophisticated applications. One such example is that of Microsoft’s Tay,7 a conversational bot whose training continued with Twitter data, leading it to post offensive and racial tweets. It had to be shut down after only 16 hours of service. Clearly, AI strategy must carefully articulate how companies will address these data management and design challenges. Note that some data-related problems manifest from more systemic organizational issues, such as lack of cross-functional communication or the presence of an hierarchical structure. In such cases, AI adoption may require considerable organizational change.
Infrastructure
AI strategy should also consider infrastructure-related issues. Specialized hardware and software may be required to run AI and ML models effectively. Depending on the level of sensitivity of the data, organizations may decide to have an on-premise data infrastructure. For instance, some hospitals and hedge fund management companies are still averse to on-cloud infrastructure. While this option may provide the most control of the data and computing assets, it places the burden of architecting, developing, protecting, and managing infrastructure assets on the organization. Therefore, unless the nature of the data is very sensitive or the company intends to build new businesses that are entirely driven by AI capabilities, it may not be necessary to make such massive investments in infrastructure.
Algorithms
The most technical part of an AI strategy is the selection and design of the AI algorithm. However, the choice of AI algorithms depends significantly on the given use case. Most AI product development organizations build multiple models leveraging different algorithms before choosing the optimal solution.
From a theoretical standpoint, AI algorithms can either be classical or data-driven. Traditional algorithms follow a rule-based approach with deterministic outcomes, while data-driven algorithms have a range of possibilities with probabilistic outcomes. In other words, while classical algorithms produce the same result every time, the ML algorithms could produce different results for the same input depending on the data used for training. Such algorithms learn from the data and typically acquire all the biases present in the data. Over time, as AI algorithms evolve to become more sophisticated, they may also generate bias due to issues of overfitting. Addressing such concerns is among the most significant challenges in AI product development.
Ethical Considerations
Several ethical issues arise during the development of AI products. Customer privacy–related issues, job displacement, inequitable distribution of wealth, and unfair decisions due to bias in algorithms are some notable examples. While new regulations are being created to deal with these emerging issues, organizations should place customer interests and well-being ahead of the company’s interest.
How Should We Pursue the Implementation of AI Initiatives?
Most experts advise adopting a “minimum viable model” approach to the implementation of AI initiatives. Such a paradigm can be applied to both the development of the underlying AI/ML models and the platform on which these models run. For instance, if an organization is in the business of forecasting home prices, it could develop a baseline model using multiple regression. This model may have slightly higher error rates than is ideal, but in-the-ballpark forecasts are available. In the next iteration, the model can be improved using nonlinear regression. Subsequently, time series analysis can be performed to enhance the model even more. This approach ensures that the baseline model begins to deliver business value as soon as possible. On successful validation of the use case from a business perspective, the model is continuously refined to produce the best results.
Similarly, organizations should shy away from building massive infrastructure, comprising sophisticated hardware (e.g., GPUs) and platforms such as data lakes, before establishing the use case. Instead, they should follow a minimum viable model regarding platform. In this approach, they build their product on a plug-and-play AI platform. They can also leverage cloud environments such as Amazon AWS, Microsoft Azure, or Google Cloud to create AI services. After establishing the use case and validating the market need for their AI initiative, they can make more investments in infrastructure.
The gradual approach to the adoption and implementation of AI initiatives makes the most practical sense. As Figure 2 shows, some AI initiatives can be implemented quickly by integrating with AI services of a third-party platform, while others require long cycles of development, training with data, and comprehensive testing. Chatbots, text-to-speech, and automation of rudimentary tasks are some instances of low-hanging fruit that can generate some quick wins. Organizations can then develop more involved use cases with huge business potential. Examples of such involved cases are computer vision and computer-aided diagnosis. Finally, companies can develop new business ideas and business models that rely on AI (self-driving cars is one example).
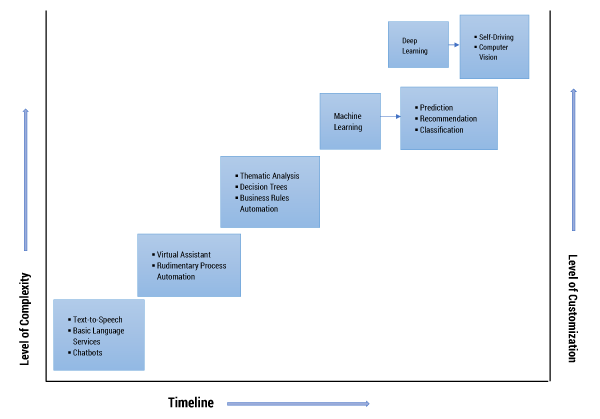
Some Best Practices to Help Organizations Implement AI Initiatives
Know That Digital Maturity Is Key
An Agile mindset, iterative development cadences, cross-functional collaboration, and metrics-driven measurement are some organizational factors used in assessing digital maturity. Organizations may have to reskill and upskill some employees to achieve the desired process maturity of the company and may need to hire individuals with AI, ML, and digital experience. Digital maturity is developed over a continuum and is not an all-or-nothing exercise. Therefore, all efforts made in developing a digital enterprise help facilitate a smoother AI adoption.
Expect Early Setbacks
Initial failures and setbacks are quite common. However, high rates of failure should not deter organizations from pursuing AI opportunities. Instead, organizations should focus on achieving strategic clarity. They should work toward testing business hypotheses efficiently by failing fast and cheap and avoiding unnecessary spending. Such a lean mindset is crucial, as even failed initiatives help build digital maturity and experience in dealing with the challenges AI presents, which could help companies find eventual success.
Develop Good Training Data
Organizations typically require robust data management for AI initiatives to be successful. The whole process of data collection, cleansing, transformation, and loading is necessary. However, a more subtle challenge is the process of reducing bias in data. Training data is the major determinant of the actions and behaviors of AI products and services. In other words, data in AI applications plays the same role that functional specification does in traditional information systems. The development of training data is a critical step but is also the weakest link in the chain of activities. Most projects fail not because of poor algorithm design or unskilled AI engineers but because of a lack of proper training data sets.
Along with data engineers, it is typically the responsibility of product managers and data governance teams to ensure that the AI development team has access to high-quality training data. It is imperative that subject matter experts and product managers who possess comprehensive understanding of the business domain determine the relevant training data. Their choice should be based on the business rules and must be representative of the underlying data generated by business transactions. Delegating such crucial decisions to implementors may lead to unintended consequences stemming from lack of good-quality training data.
Expect Organization-Wide Impact
AI initiatives require data from multiple sources within the company, meaning that organizations will need to build a knowledge base of corresponding workflows, business processes, and nuances across business domains such as regulations or custom workflows. This effort requires collaboration among several cross-functional units, which can be daunting, especially for traditional companies used to working in functional silos. Traditional companies usually face higher hurdles when middle management (typical power centers within the organization) feels challenged. In such cases, AI initiatives might turn into comprehensive change management and organizational transformation projects.
Recognize That Leadership Is Critical
While AI interventions are vital to an organization’s success, they often raise several ethical issues. One such issue is AI’s potential for mass job displacement, which is a source of anxiety for operational staff as well. Leaders should reassure their employees about job safety and take steps to reskill their employees. Such leadership will encourage all employees within the company to aggressively leverage AI capabilities in their day-to-day work to achieve sustained competitive advantage for the organization.
Expect Strict Regulations
Technology enterprises should expect more regulatory hurdles, especially around the privacy of user data. The European Union’s General Data Protection Regulation (GDPR) and the California Consumer Privacy Act (CCPA) in the US are already in place; other US states intend to pass rules similar to California’s. AI and data-oriented companies may face considerable challenges due to a lack of regulatory clarity as these regulations continue to evolve. Digital organizations need to place special emphasis on cybersecurity, data-specific audits, and regulatory compliance.
Many pioneering companies are exploring federated learning approaches to mitigate these emerging risks. Federated learning is a ML approach where statistical models are executed over remote devices, keeping data local to those remote devices or data centers. Such distribution of data across several remote sources acts as an effective control by preventing access to the whole data set in the event of a security attack.
Conclusion
This article highlights multiple salient facets of AI strategy and adoption planning. A colloquial expression that best captures the current AI market situation is, “The genie is out of the bottle.” It is in the best interest of organizations to leverage the “genie” to their advantage by becoming early adopters — but we urge organizations to tread the path with caution. A gradual but consistent approach to AI initiatives should help organizations compete effectively in these early phases of the AI revolution.
References
1Hall, Curt. “AI & Machine Learning in the Enterprise, Part I: Current Status.” Cutter Consortium Data Analytics & Digital Technologies Executive Update, Vol. 18, No. 2, 2018.
2Dans, Enrique. “Artificial Intelligence, Buzzwords and Competitive Value.” Forbes, 25 September 2017.
3We reviewed contemporary literature to develop a deeper understanding of state-of-the-art thinking on strategic considerations in AI product development. We also conducted multiple semistructured interviews with seven subject matter experts in the field, including two AI product managers, two founders of an AI company, two AI engineers, and one researcher. We discussed the following three broad topics in interview sessions with each expert: (1) What is an AI strategy? (2) What is the process of developing an AI strategy? and (3) What are the fundamental components of an AI strategy?
4Cristianini, Nello. “The Road to Artificial Intelligence: A Case of Data over Theory.” New Scientist, 26 October 2016.
5Gohil, Raveena. “Artificial Intelligence and Machine Learning: How Are They Different?” ACE (blog), 13 August 2019.
6“Worldwide Spending on Cognitive and Artificial Intelligence Systems Forecast to Reach $77.6 Billion in 2022, According to New IDC Spending Guide.” IDC, 19 September 2018.
7Kastrenakes, Jacob. “Microsoft Made a Chatbot That Tweets Like a Tee.” The Verge, 23 March 2016.